🔺 Edge#146: A Deep Dive Into Arize AI ML Observability Platform
This is an example of TheSequence Edge, a Premium newsletter that our subscribers receive every Tuesday and Thursday. On Thursdays, we do deep dives into one of the freshest research papers or technology frameworks that is worth your attention.
💥 What’s New in AI: A Deep Dive Into Arize AI ML Observability Platform
In Edge#145, we covered Arize AI as one of the early pioneers in the area of ML observability. Today, we would like to deep dive into the core capabilities of the Arize AI platform and their applicability in ML pipelines.
Arize AI is a platform designed to enable end-to-end observability in ML pipelines. The platform is designed to provide model observability capabilities across training, validation, and production environments, working with different ML platforms and frameworks. Additionally, you are likely to find Arize AI integrated with mainstream ML stacks such as Azure ML, Google Cloud ML, and Azure ML.
Capabilities
From a functional standpoint, Arize AI provides more than just ML observability capabilities but observability is definitely the area where it excels. While you might find a wide overlap between Arize AI and other ML monitoring platforms, the key difference is that Arize AI goes a step beyond monitoring to infer the root cause of performance changes in ML models. At a high level, the Arize AI platform performs statistical validations across the different elements and stages of the lifecycle of ML models ranging from feature inputs to model outputs.
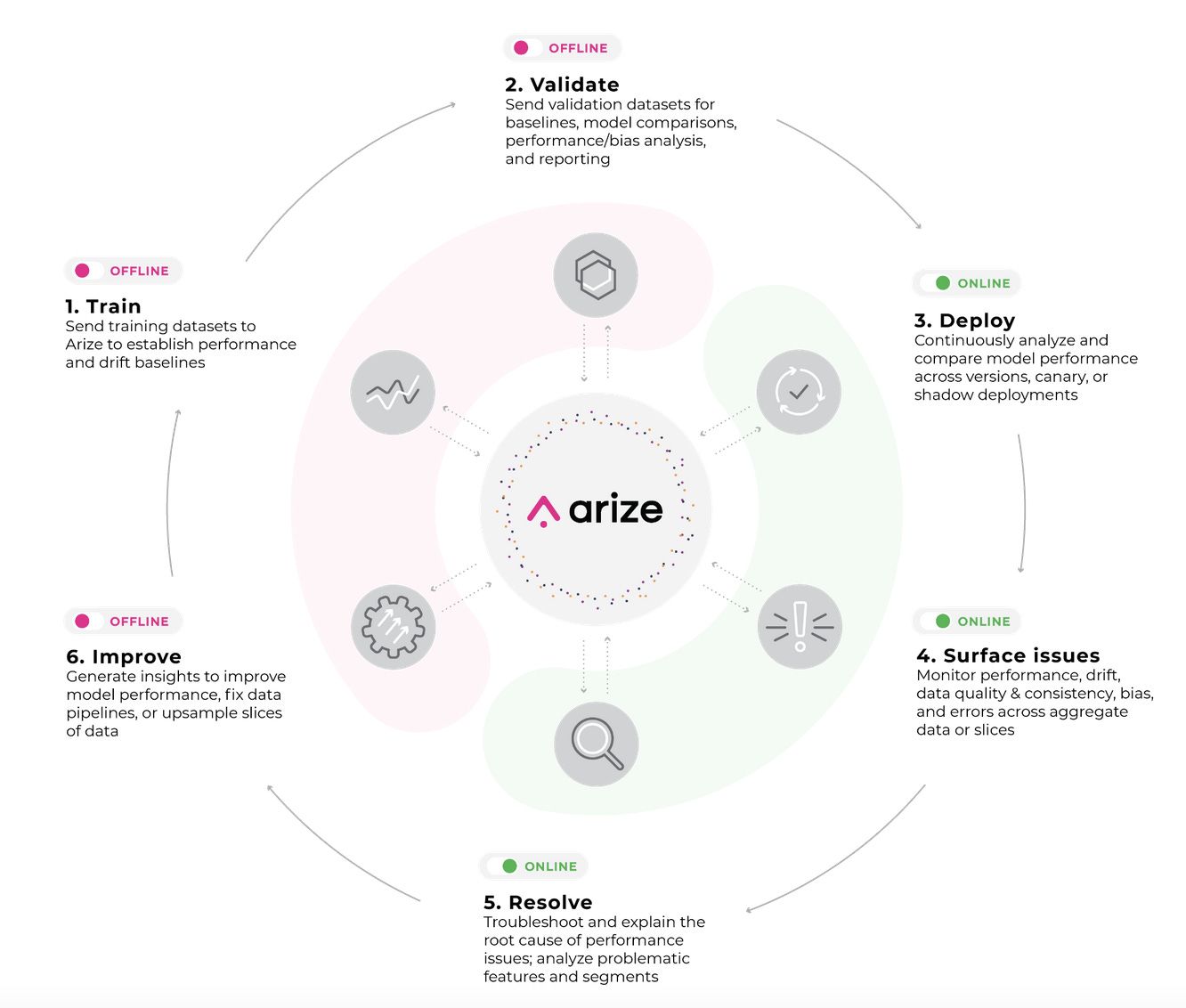
By enabling statistical checkpoints across the data and model inputs and outputs, Arize AI enables some key capabilities that constitute the foundation of observability in ML pipelines:
Model Lineage, Validation & Comparison: Arize AI has pre-launch validation capabilities as well as model versioning and lineage support that are helpful in comparative analysis.
Performance Monitoring & Root Cause Analysis: Arize AI offers end-to-end monitoring and visualizations that help get to the bottom of model failures and performance degradations. Standard model performance metric monitoring is enhanced with additional functionality, like production A/B testing of models.
Drift Analysis: Arize AI provides cohort analysis of concept, model and data drift. The platform also allows users to see the impact of drift on model performance on the same chart.
Data Quality: Arize AI monitors data quality, consistency, and anomalous behavior on predictions across the lifecycle of ML models. The platform also offers the ability to monitor for data consistency between offline and online data streams.
Explainability: Arize AI offers the ability to view feature importance for the top n features; it also supports global/local/cohort feature importance — all without requiring a model upload.
Fairness and Bias: Arize AI tracks fairness and bias indicators across the lifecycle of ML models.
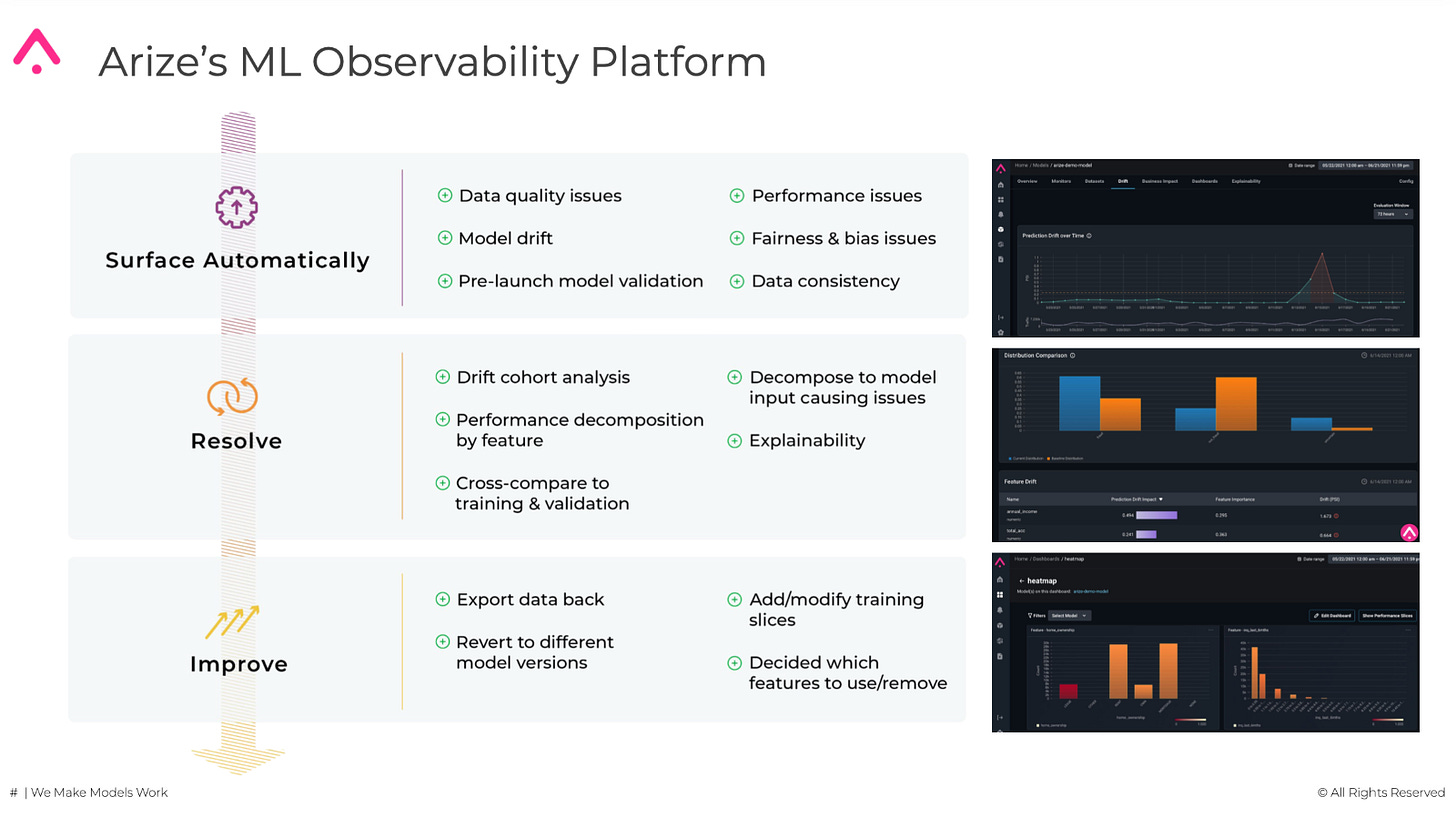
One reason Arize AI’s ML observability platform stands out in a crowded field is its ability to automatically surface up performance issues by feature, value, and cohort. Arize AI lets users click directly into low-performing slices (feature/value combinations) for root cause analysis rather than requiring users to spend time digging into SQL to surface problems. Other differentiators of Arize AI include an architecture built to handle analytic workloads across billions of daily predictions, model versioning and lineage support to track and compare models across the ML lifecycle, and the ability to support business impact analysis.
Another benefit is that its capabilities are platform agnostic and can be leveraged from different ML technology stacks whether on-premise or cloud-based. Arize AI can be natively used in over a dozen ML runtimes including platforms such as AWS Sage Maker, Google Cloud ML, Azure ML, Databricks, Ray, and many others. The platform also provides first-class integration with different components of the lifecycle of ML models such as feature stores like Feast, hyperparameter optimization stacks like Weights&Biases, or notebook environments such as DeepNote.
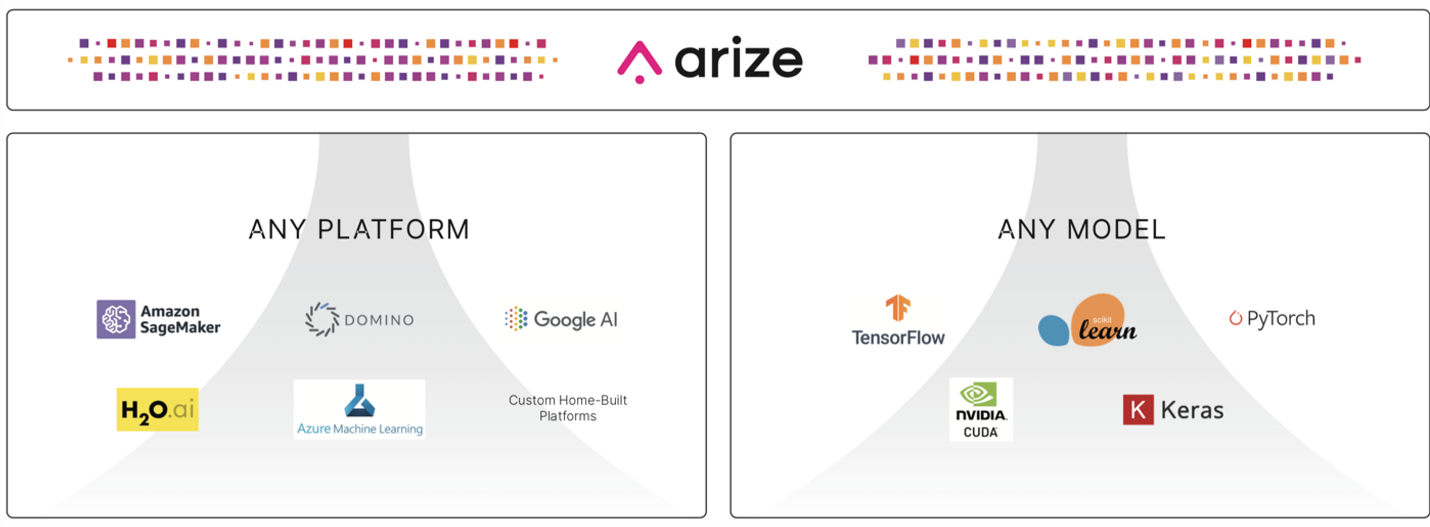
Using Arize AI is relatively straightforward, the data scientist can start by injecting a few lines of code into their ML model to log the relevant information. After that, we can use the Arize AI dashboard to configure the appropriate monitors and dashboard to analyze the performance of the model and related datasets.
The Evaluation Store
A key innovation in the Arize AI platform is the introduction of the Evaluation Store concept. You can think about this component as an extension of a feature store that focuses on validating, monitoring, and improving model performance. Additionally, Arize AI’s evaluation store provides clear model lineage and performance analysis as well as comparison across different model versions and datasets. Even more relevant is the fact that an evaluation store can provide continuous feedback about the performance of models.
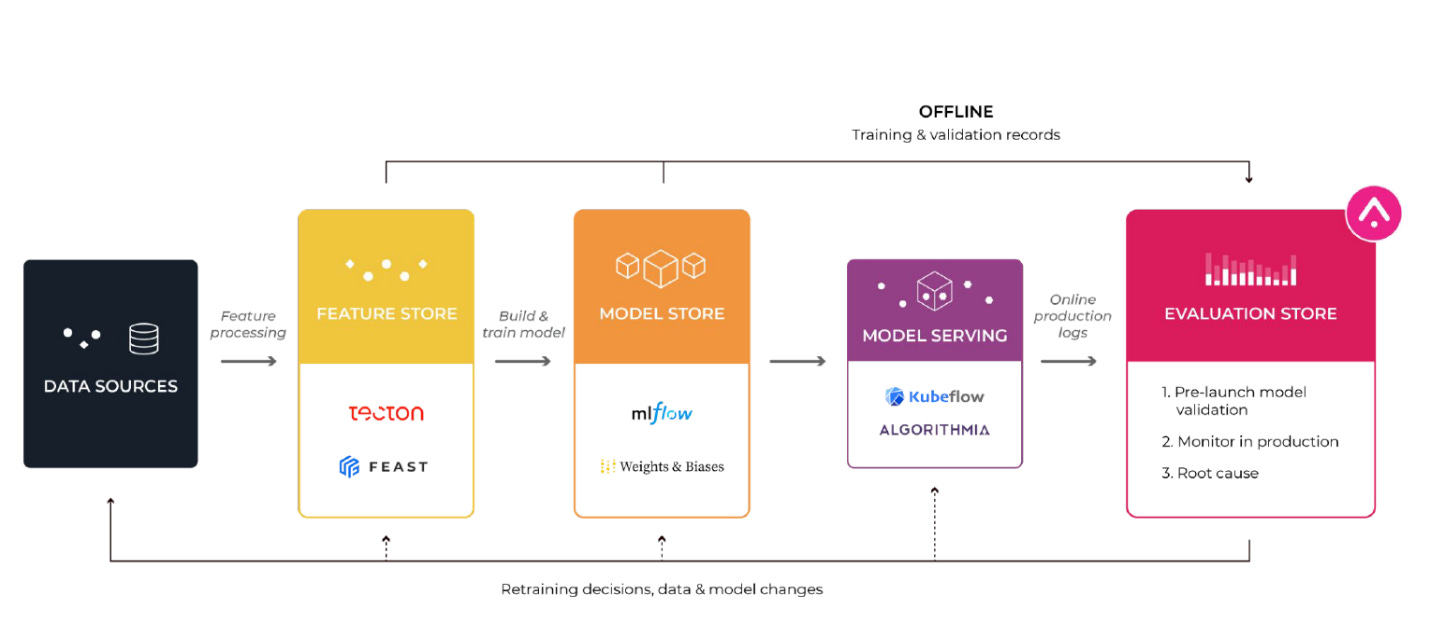
Conclusion
Observability is one of the emerging trends in the ML ecosystem. While the number of platforms that incorporate observability as a native capability is still small, the relevance of this feature in ML applications has been progressively increasing. Arize AI is one of the pioneers in the field of ML observability. Building on innovative concepts such as the evaluation store, Arize AI provides ML monitoring and observability capabilities in a platform-agnostic model that can be integrated into many deep learning frameworks and technology stacks. From simpler feature impact analysis to sophisticated model explainability and root cause inference capabilities, Arize AI provides a fairly comprehensive feature set to incorporate observability into your ML solutions in a non-disruptive way.
*We’ve partnered with Arize AI to present you a live product demo (12/15) and a webinar on ML observability in lending featuring a fireside chat with America First Credit Union (12/8). Check the links to learn more.