Decentralized AI: Myth or Reality?
Weekly newsletter that discusses impactful ML research papers, cool tech releases, the money in AI, and real-life implementations
📝 Editorial
The next two issues of TheSequence Edge will cover the emerging topic of decentralized artificial intelligence (AI). Research in the area of decentralized AI is certainly nascent and its technologies are far from seeing mainstream adoption. However, this trend has the potential of becoming largely influential in the next few years of the AI industry. In principle, decentralized AI aims to remove central control from the lifecycle of machine learning (ML) models. Imagine a scenario in which multiple parties collaborate with different datasets to train a given ML model, without delegating complete control onto any one of them.
The relevance of decentralized AI is being catalyzed by two fundamental factors. On one side, we have the emergence of in-device ML models in mobile and internet of things (IoT) scenarios that can operate without centralized server-side control. Additionally, there is an increasing concern that the centralized nature of AI solutions is increasing the gap between the large technology platforms, such as Google, Facebook, Amazon and Apple, and the rest of the world. These two factors, combined with a series of technological innovations, have propelled the first generation of decentralized AI technologies, introducing very active research in the space.
We will deep dive into this topic next week. Be prepared to learn a lot, the information about Decentralized AI is scarce.
🔺🔻TheSequence Scope – our Sunday edition with the industry’s development overview – is free. To receive high-quality educational content every Tuesday and Thursday, please subscribe to TheSequence Edge 🔺🔻
🗓 Next week in TheSequence Edge:
Edge#35: the concept of decentralized AI; Microsoft Research paper on decentralized ML models using blockchains; SingularityNet’s decentralized AI platform.
Edge#36: Blockchains, Smart Contracts and Incentives in Decentralized Machine Learning; a follow-up paper on decentralized ML models using blockchains; Ocean Protocol, a decentralized platform for data sharing.
Now, let’s review the most important developments in the AI industry this week.
🔎 ML Research
Google Performer
Google Research published a paper introducing Performer, an optimized transformer architecture that can scale linearly ->read more on Google Research blog
Interpretability vs. Learning
Facebook AI Research (FAIR) published three research papers providing a new perspective of the friction between interpretability and learning in deep learning models ->read more on FAIR blog
PowerTransformer
Researchers from the Allen Institute of AI published a paper introducing PowerTransformer, a model to identify and correct characters in a narrative ->read more in the original research paper
🤖 Cool AI Tech Releases
PyTorch 1.7
The new release of PyTorch is generally available. The new framework includes cool capabilities such as support for CUDA 11 architecture and notable improvements to its vision library ->read more on PyTorch blog
Microsoft Lobe
Microsoft released Lobe, an app that enables the training of machine learning models without writing any code ->read more Microsoft AI blog
mT5
Google open-sourced mT5, a model that can achieve state-of-the-art performance in language tasks across 101 languages->read more on mT5’s GitHub page
💬 Useful Tweet

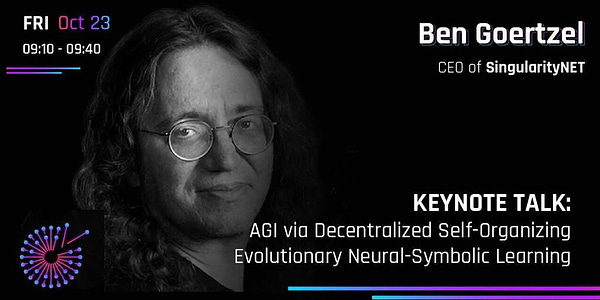
💸 Money in AI
AI-powered website data analytics startup SimilarWeb has raised $120 million. Using machine learning algorithms and deep learning, they analyze vast amounts of data from different sources for important signals and insights about one’s website and its competitors.
Real-time analytics startup Rockset raised $40 million in Series B funding. Data hungry areas such as real-time personalization and recommendation engines, real-time supply chain logistics and delivery tracking systems, gaming leaderboards, fraud detection systems, health and fitness trackers, social media news feeds and others, demand high-performance search and analytics at scale. Rockset provides it, building a converged index on all types of data: structured, semi-structured, geographical and time-series data.
Intelligent visual assistance startup TechSee raised a $30 million investment round. Using augmented reality and computer vision, it allows customers to independently resolve a wide variety of issues that cannot be handled by traditional "blind" chatbots. By pointing their smartphone at the equipment and allowing the real-time recognition of the device, clients get step-by-step visual and conversational guidance to solve the issue.
Site-specific agricultural data analytics startup Arable raised $20 million in a funding round. The startup combines data from thermal and acoustic sensors, satellite imagery, observed conditions with historical data to create AI-driven forecasts. For example, they leverage deep learning with audio to monitor rainfalls, and generative adversarial networks (GANs in Edge#8) to generate synthetic, plausible seasons for growers, particularly the management schedule, for the recently acquired land.
Consumer-controlled health data startup Human API raised $20 million in funding. Leveraging inference models personalized to individual profiles, Human API converts unstructured data into a consistent format (e.g., PDF, CSV) that’s enriched with industry-standard coding systems.
Water analytics startup Ketos raised $18 million in funding. The startup monitors data from the sensor called Shield Fabric and analyzes it with ML models to flag toxins. It also provides historical context to better water management, process optimization, and advanced diagnostics for maintenance-related predictions. With more data amassed, the startup claims that it can study the health impacts of water across ZIP codes.
AI-enabled cognitive technology startup platform Skan.ai raised $14 million in a funding round. The startup combines computer vision, deep learning, and machine intelligence to observe and optimize business processes, uncovering how workloads are performed in an organization.
AI-powered helpdesk Capacity raised $11 million in Series C financing. ML algorithms power Capacity’s natural language processing to learn from organizational knowledge and business interactions, helping automate clients’ helpdesk, processes and decisions in real-time.
AI model optimization platform Deci has raised a $9.1 million seed round. Harnessing AI-based algorithmic development capabilities, Deci’s deep learning platform automatically gears up neural networks and makes it easier and faster for businesses to take AI workloads into production, optimizing the production models for improved accuracy and performance along the way.
Privacy-preserving data engine DataFleets raised a $4.5 million seed round. The startup helps data scientists access and analyze data without compromising privacy. It uses an approach called federated learning (Edge#5), where instead of bringing the data to the model, they bring the model to the data.
This is a free Sunday TheSequence Scope. For the full experience, become a paying subscriber for TheSequence Edge. Thank you for your support.
TheSequence is a summary of groundbreaking ML research papers, engaging explanations of ML concepts, exploration of new ML frameworks, and platforms. It also keeps you up to date with the news, trends, and technology developments in the AI field.
5 minutes of your time, 3 times a week – you will steadily become knowledgeable about everything happening in the AI space.
