🔶 The Difficult Economics of AI Companies
Weekly newsletter that discusses impactful ML research papers, cool tech releases, the money in AI, and real-life implementations
📝 Editorial
AI is the most important trend in modern technology and, as a result, we are witnessing an explosion of startups in the space. However, unlike other technology trends, AI startups face very different economics in order to be able to scale. In general, AI startups are more capital intensive than their equivalent in previous technology trends. There are a few factors contributing to this phenomenon. For starters, AI technologies require high-quality datasets in order to perform and those are relatively expensive to get. Additionally, there is also a cost barrier in order to attract top data science talent, which is really scarce. However, the main challenge for AI startups comes from incumbent technology companies.
For decades, we’ve seen many technology trends evolve following Clayton Christensen’s innovators’ dilemma thesis, in which startups disrupt incumbents that are usually slower to move into the new market. That’s not happening in AI, quite the opposite. The research labs from companies like Google, Microsoft, Uber, Facebook, and Amazon are actively driving as much, if not more, innovation in the AI space as the current generation of startups. Those software giants are also highly active in acquiring young AI startups in order to capture top data science talent. Many people consider the dynamics of the AI market as the antithesis of the innovator’s dilemma market structure. One thing is certain, the economics of AI startups are tricky and different from anything we have seen before.
🔺🔻TheSequence Scope – our Sunday edition with the industry’s development overview – is free. To receive high-quality educational content every Tuesday and Thursday, please subscribe to TheSequence Edge 🔺🔻
🗓 Next week in TheSequence Edge:
Edge#17: the concept of Bayesian Neural Networks; a method created by DeepMind to use Bayesian Neural Networks to assess the fairness of a dataset; Pyro, a probabilistic programming language created by Uber.
Edge#18: the concept of Production-Ready notebooks; Microsoft research paper about the challenges with computational notebooks; Polynote, a next-generation notebook platform created by Netflix.
Now, let’s review the most important developments in the AI industry this week.
🔎 ML Research
Understanding Transfer Learning
Google AI researchers published a paper that explores some ideas about what makes transfer learning work ->read the research paper here
Advancing Embodied AI
Embodied AI looks to build agents that understand the physical environments around them. Facebook AI Research (FAIR) published a compendium of several papers about new developments in the area ->read more on the FAIR team blog
🤖 Cool AI Tech Releases
LinkedIn Fairness Toolkit (LiFT)
LinkedIn open-sourced its fairness toolkit, a Scala/Spark library that enables the measurement of fairness in large scale machine learning workflows ->read more on GitHub
Facebook Submitit
Facebook AI Research open sourced Submitit, a framework for submitting Python computation jobs, such as the ones used in machine learning models, to high scalable Slurm clusters ->read more on FAIR team blog
Neuralink
Elon Musk demonstrated a pig with an implanted brain device, developed by his neural engineering startup Neuralink. The device works as a digital link between brains and computers. Currently, it only shows brain activity. In the long term, it's supposed to be able to interpret it and communicate the signals back from computers ->watch the whole presentation here or ->watch the cut version from CNET
💬 Useful Tweet
An enterprise newsletter from VC powerhouse Andreessen Horowitz includes an insightful post discussing models to improve the economics of AI startups

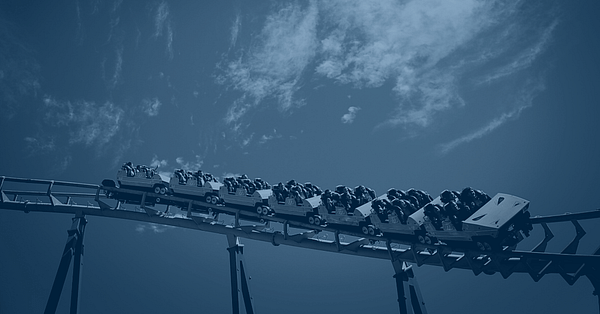
💸 Money in AI
The White House announced a $1 billion investment into AI and quantum computing. NSF’s AI Research Institutes and DOE’s QIS Research Centers will serve as national R&D hubs to work on foundational problems and “pursue transformational advances” across topics like quantum computing, machine learning and computer vision.
The Vector Institute dedicated to advancing AI established a team to commercialize its industrial and health care research.This independent nonprofit was launched in March 2017 with $135 million in total funding over five years from the government of Canada, the government of Ontario, and almost 40 Canada-based companies.
Synthego, a startup that provides machine learning-based full-stack genome engineering solutions, has just raised $100 million. Combining automation, machine learning, and gene editing, the solutions significantly accelerate the R&D process.
ProteanTecs, a startup that applies AI to chip design and its reliability monitoring, raised $45 million in a round of funding.
Digital ID verification startup Socure closed a $35 million round. To increase auto-approval rates, reduce false positives and capture more fraud, its machine learning algorithms and predictive analytics techniques tuned to authenticate people from “thousands” of sources like email, phone, address, social media, and other IP data points. The same week another ID verification startup, Berbix, also raised funds – $9 million.
MLOps software startup Vertaraised a $10 million in Series A. The team works on bridging the gap between data scientists and developers to accelerate the deployment of machine learning models. Verta’s platform allows users to track, deploy, operate and monitor models with production-grade MLOps tools.
This is a free Sunday TheSequence Scope. For the full experience, become a paying subscriber for TheSequence Edge.
TheSequence is a summary of groundbreaking ML research papers, engaging explanations of ML concepts, exploration of new ML frameworks, and platforms. It also keeps you up to date with the news, trends, and technology developments in the AI field.
5 minutes of your time, 3 times a week– you will steadily become knowledgeable about everything happening in the AI space.

Nice piece!..thanks for the resources also provided on the page..very insightful..especially the research paper on transfer learning..