π Guest post: Using AI to Learn a Disentangled Gait Representation for Versatile Quadruped Locomotion*
The Oxford Robotics Institute (ORI) is built from collaborating and integrated groups of researchers, engineers and students all driven to change what robots can do for us. The ORI is interested in a diverse range of robotics, from flying to grasping, inspection to running, haptics to driving and exploring to planning. This spectrum of interests leads to research across a broad span of topics, including machine learning and AI, computer vision, fabrication, multispectral sensing, perception and systems engineering. In this condensed article, the ORI team investigates a new approach to stabilizing robotic walking - the full study can be read here.
Project Background
Quadruped locomotion is rapidly maturing to a degree where robots now routinely traverse a variety of unstructured terrains. However, while gaits can be varied typically by selecting from a range of pre-computed styles, current planners are unable to vary key gait parameters continuously while the robot is in motion. The synthesis of βon-the-flyβ gaits with unexpected operational characteristics or even the blending of dynamic maneuvers lies beyond the capabilities of the current state-of-the-art. ORI attempt to address this limitation by learning a latent space capturing the key stance phases of a particular gait, via a generative model trained on a single trot style. The use of a generative model facilitates the detection and mitigation of disturbances to provide a versatile and robust planning framework. ORI evaluates its approach on a real ANYmal quadruped robot and demonstrates that their method achieves a continuous blend of dynamic trot styles while being robust and reactive to external perturbations.
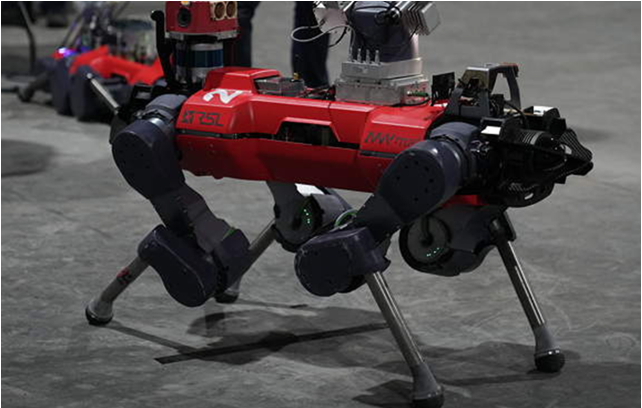
Quadruped locomotion has advanced significantly in recent years, extending its capability towards applications of significant value to industry and the public domain. Driven primarily by advances in optimization-based and reinforcement learning-based methods, quadrupeds are now able to traverse over a wide variety of terrains, making them a popular choice for tasks such as inspection, monitoring, search and rescue or goods delivery in difficult, unstructured environments.
Designing a New Approach
Inspired by recent work, ORI approached the challenge of continuous contact-schedule variation from the perspective of learning and traversing a structured latent-space. This is enabled by learning a generative model of locomotion data which, in addition to capturing relevant structure in the space, enables the detection and mitigation of disturbances to provide a versatile and robust planning framework. In particular, ORI train a variational auto-encoder (VAE) on short sequences of state-space trajectories taken from a single gait type (trot), and predict a set of future states.
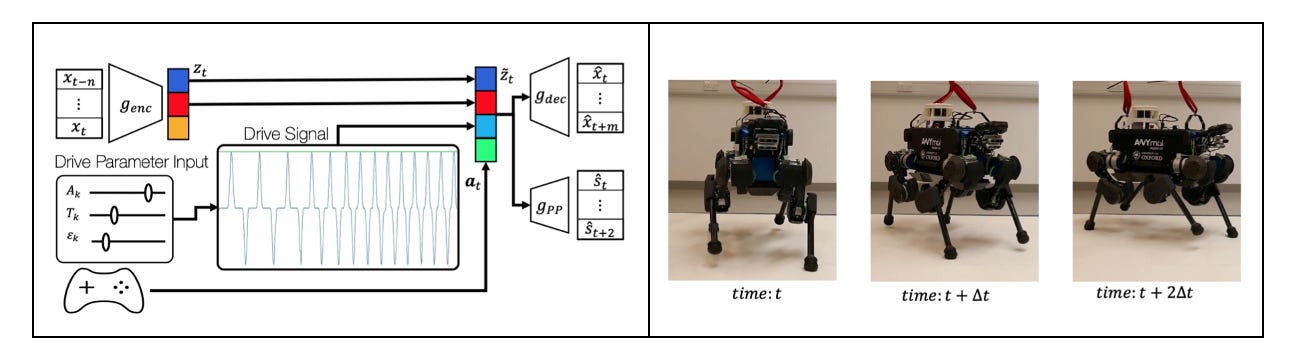
The VAE is fast enough to act as a planner in a closed-loop controller, so it can react to external disturbances and mitigate against real-world effects such as un-modeled dynamics and hardware latency. For closed-loop control, ORI began by encoding a history of robot states from the raw sensor measurements to infer the current gait phase, storing a buffer of past robot states to create the encoderβs input. Therefore, any disturbances are characterized as out of distribution with respect to the training set. Given the generative nature of this approach, this discrepancy is quantified during operation by the trained model via the Evidence Lower Bound (ELBO).
Demonstrating Enhanced Stability
The ELBO is used to detect disturbances as it is a lower bound for the evidence of a sample given a particular distribution. The distribution in question is that learned over the training data, therefore, any motions which deviate from this due to a perturbation cause a large negative spike in the ELBO value.
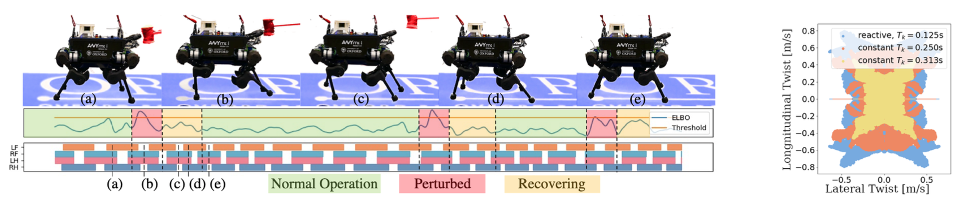
In Summary
ORI presented a robust and flexible approach for locomotion planning via traversal of a structured latent-space, utilizing a deep generative model to capture features from locomotion data and enable detection and mitigation of disturbances. Utilizing a generative model affords detection of disturbances as out of the distribution seen during training. The VAE-planner is able to reject a wide range of impulses applied to the robotβs base. This operating window is enlarged by increasing the robotβs cadence once a disturbance is detectedβa rudimentary response, corresponding to how humans increase their cadence to recover from slippage.
ORI trained its models on a hardware cluster of six NVIDIA DGX A100 servers combined with a multi-GPU NVIDIA RTX 6000 server and a PNY 3S-2400 storage array. This cluster is part of Scan Computersβ Cloud service, provisioned with Run:ai Atlas software in order to virtualize the GPU pool across the DGX nodes to facilitate maximum utilization. Run:ai Atlas also provides a mechanism for scheduling and allocation of ORI workflows across the cluster.
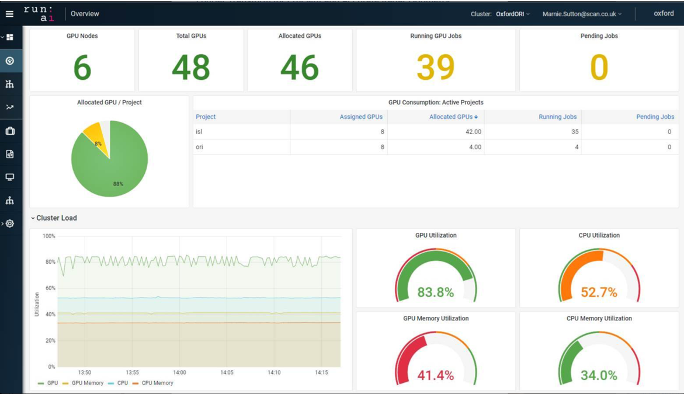