👾Transformers are Getting More Ambitious
Free news digest about the most important things that happen in the ML world
📝 Editorial
Recently, we started a new series at TheSequence covering the intricacies of transformer architectures. Considered by many the most important development in the recent years of deep learning, transformers revolutionized language intelligence. Models such as Google’s BERT and OpenAI’s GPT-3 have laid out the path for new highs in natural language processing (NLP) models. Despite all the success, constraining transformers to NLP scenarios would be a mistake. These days transformers are getting more ambitious and are applied to all sorts of deep learning scenarios.
It turns out that the same attention mechanisms that make transformers so effective for language models can be used in other domains. Building on the NLP breakthroughs, transformers have found tremendous success in computer vision scenarios. Facebook has recently published some amazing work applying transformers and self-supervised learning to computer vision models. But researchers are not stopping there. Just this week, DeepMind and Microsoft published their work in cutting-edge applications of transformer models. Microsoft unveiled details about their work using transformer models to improve Bing search scalability and accuracy. DeepMind’s work seems even more ambitious adapting transformers to build models that can process different types of inputs such as text, image, audio, and many others. This trend is likely to continue and we should see more regular efforts expanding transformer models into scenarios beyond language. Everything indicates that the transformers are going to remain very relevant to the near-term future of deep learning.
🔺🔻TheSequence Scope – our Sunday edition with the industry’s development overview – is free. To receive high-quality content about the most relevant developments in the ML world every Tuesday and Thursday, please subscribe to TheSequence Edge 🔺🔻
🗓 Next week in TheSequence Edge:
Edge#113: the concept of Google BERT; Google TAPAS that can query tabular data sets using natural language; AutoNLP – a new beta project from Hugging Face to train and deploy transformer-based models for different tasks.
Edge#114: AI2’s Longformer – a transformer architecture optimized for the processing of long-form texts.
Now, let’s review the most important developments in the AI industry this week
🔎 ML Research
Perceiver IO
DeepMind announced the paper and code for Perceiver IO, a generalized version of Perceiver architecture, that now can scale to large and diverse inputs and outputs and deal with many tasks or types of data at once ->read more on DeepMind blog
Make Every feature Binary (MEB)
Microsoft published a detailed blog post about MEB, a large-scale sparse model that is trained on more than 500 billion query/document pairs to complement Transformer models and improve search relevance ->read more on Microsoft blog
Progressively Better Language Models
Amazon Research published a paper detailing a technique to ensure that language models don’t regress over time ->read more on Amazon Science blog
🛠 Real World ML
Building The Wall
The Airbnb engineering team published an insightful blog post about the challenges of adding data checks at scale and how it motivated them to create the Wall Framework to prevent data bugs company-wide ->read more on Airbnb engineering team blog
Quality Issues for Large-Scale Datasets
The Uber engineering team published a comprehensive blog post about how they created the data quality standards and built a cross-functional data quality platform to ensure flawless operations ->read more on Uber blog
🤖 Cool AI Tech Releases
PyTorch Profiler 1.9
PyTorch released a new version of its profiler tool, optimized to assess the performance of distributed ML models ->read more in the post by the PyTorch team
Droidlet
Facebook AI Research (FAIR) open-sourced droidlet, a robotic development platform that simplifies integrating computer vision and language models in embodied systems and robotics to facilitate rapid prototyping ->read more on FAIR blog
TimeDial and Disfl-QA
Google Research open-sourced TimeDial and Disfl-QA, two datasets optimized for different NLP tasks ->read more on Google Research blog
VoxPopuli
To accelerate advanced NLP systems across the globe, FAIR released VoxPopuli, a massive multilingual speech data set that provides 400,000 hours of unlabeled speech data in 23 languages ->read more in the original paper
💬 Useful Tweet
We explain the concepts and relevant research papers in our regular ML threads.

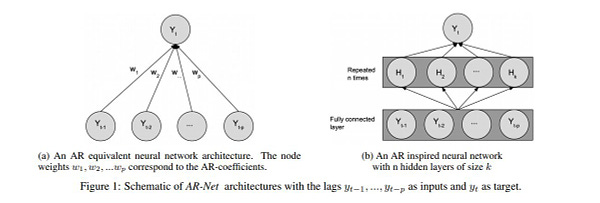
💸 Money in AI
Building AI&ML:
Data science platform Dataiku raised a $400 million Series E at 4 billion evaluation. Tiger Global led the round. Hiring on positions from A to V.
Deep Genomics that created AI Workbench platform to decode vast amounts of data on RNA biology raised $180 million in Series C funding led by SoftBank Vision Fund 2. Many interesting positions.
Conversational AI platform provider Yellow.ai raised $78.15 million in a Series C round led by WestBridge Capital.
AI chatbot startup Heyday was acquired by Hootsuite in a $60 million CAD deal. 25 open job positions.
Cloud data lake services provider Ahana raised $20 million in a Series A round led by Third Point Ventures. Hiring in the US and remote.
Speech recognition company Deepgram launches a $10 million startup program. It gives up to $100,000 credits to eligible startups to create, launch, and scale voice-enabled experiences with speech recognition. You can apply here.
You have talked about Longformer in the #edge114 & not #edge112. It took lots of time to find out this error :)