1️⃣2️⃣3️⃣ Three Data Science Trends that are Hard to Live Without in 2021
Educational newsletter that discusses impactful ML research papers, cool tech releases, the money in AI, and real-life implementation
📝 Editorial
No, this is not one of those annoying 2021 prediction write-ups so stay with us for one minute 😉
I don’t think anyone would argue that 2021 promises to be a spectacular year for the AI market in general. The more interesting question is, which areas of the market are set to experience more mainstream adoption? I try to not play the game of forecasting macro-market trends because I think there are enough people making noise about that already. Instead, I can try to relate my practical experience, both as a CEO of data science company IntoTheBlock and as an active angel investor in the machine learning space, about some trends that I wasn’t really convinced of at the beginning of 2020, those I now find hard to envision a future without.
This newsletter is mostly oriented to developers and machine learning practitioners, focusing on practical applications of data science. From that perspective, our audience cares about technology and research that can be used today instead of the super futuristic stuff. During 2020, I saw our data science teams regularly adopt several machine learning technologies to the point that, today, I find it very hard to envision any machine learning effort that doesn’t include those stacks. The list is fairly large but here are my top three:
AutoML and Neural Architecture Search: AutoML stacks are rapidly evolving and have proven to be very effective in accelerating the experimentation cycles of data science teams. I think we are likely to see interesting growth in this space.
Interpretability: Because InteTheBlock works in quant models, interpretability is a key area of focus for us. That sector of the market saw a few interesting startups achieve relevant milestones in 2020 and that trend is likely to continue in 2021.
MLOps: These days, it’s hard to envision building machine learning at scale without an MLOps stack. The innovation in this sector is off the charts and we are likely to see new companies and technologies pop up during this year.
This list is by no means exhaustive but, like everything in this newsletter, we try to keep things simple and practical. From our vantage point, those three trends should play a role in most mainstream machine learning efforts in 2021. It’s going to be interesting to see what the AI market has in stock for us this year.
🔺🔻TheSequence Scope – our Sunday edition with the industry’s development overview – is free. To receive high-quality educational content every Tuesday and Thursday, subscribe to TheSequence Edge 🔺🔻
🗓 Next week in TheSequence Edge:
Edge#51: time-series forecasting and ARIMA; architectures to accelerate time series models using AutoML by Google researchers; M3, the platform powering time series at Uber.
Edge#52: deep dive into Google Meena, a language model that can chat about anything.
Now, let’s review the most important developments in the AI industry this week.
🔎 ML Research
MuZero
Researchers from DeepMind published a paper in Nature detailing MuZero, a reinforcement learning agent that was able to master several games without knowing the rules. This was a follow up from the original MuZero paper published in 2019 ->read more on DeepMind blog
Better Offline Reinforcement Learning
Researchers from Stanford University published a paper proposing a new method for offline reinforcement learning, using latent space ->read more in the original research paper
Knowledge Graph for COVID-19 Research
AI Researchers from Amazon published a paper explaining the underlying techniques used to create the AWS COVID-19 Knowledge Graph, which organizes information about the virus ->read more on Amazon Research blog
🤖 Cool AI Tech Releases
Probabilistic Machine Learning: The Book
An early open-source release of an upcoming MIT book about probabilistic machine learning is now available ->read more in the book’s GitHub repository
💬 Useful Tweet
In case you’d like some predictions

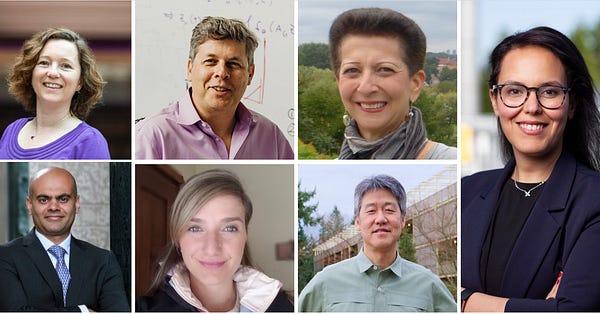
💸 Money in AI
Semiconductor startup Graphcore raised $222 million in a Series E funding round. Graphcore develops a specialized type of hardware – Intelligence Processing Units (IPUs) that hold the complete machine learning model inside the processor – to accelerate AI applications, particularly neural networks, deep learning, and machine learning.
Robotics and AI company Flexiv raised $100 million in a Series B round. Flexiv focuses on developing and manufacturing adaptive robots that integrate force control, computer vision and AI technologies.
In its annual report, AI research lab DeepMind unveils its financial situation. Its revenue has more than doubled (from $140.8 million in 2018 to $363.7 million in 2019). But the expenses grow (from $776.6 million in 2018 to $980.3 million in 2019). The company’s overall losses grew from $642.6 million in 2018 to $652.2 million in 2019. DeepMind is fully backed by Google, and they have written assurance that for at least 12 months it will continue to receive adequate financial support from Google.
Interested to be featured in TheSequence? Let us know by replying to this email.
TheSequence is a summary of groundbreaking ML research papers, engaging explanations of ML concepts, exploration of new ML frameworks, and platforms. It also keeps you up to date with the news, trends, and technology developments in the AI field.
5 minutes of your time, 3 times a week– you will steadily become knowledgeable about everything happening in the AI space.
You're right about MLOps and emerging companies! Check out ZenML, an #opensource #MLOps #framework for #reproducible #ML!
Keep the tools you love but accelerate everything around!
#GitHub: https://github.com/maiot-io/zenml
#extensible #DevOps #DL #AI #productionready #machinelearning