TheSequence Scope: Self-Supervised vs. Supervised vs. Reinforcement Learning
Initially published as 'This Week in AI', a newsletter about the AI news, and the latest developments in ML research and technology
From the Editor: Self-Supervised vs. Supervised vs. Reinforcement Learning
Traditional machine learning theory divides the world into two fundamental schools: supervised and unsupervised learning. However, we know that picture is incomplete. Reinforcement learning (RL) has been at the center of some of the most exciting developments in recent years of artificial intelligence (AI). From DeepMind’s AlphaGo beating Go’s World Champion Lee Sedol to breakthroughs in games like StarCraft or Dota2, RL systems have come the closest to show sparks of intelligence. Given that RL systems learn by interacting with an environment instead of just being trained like supervised models, many experts believe that they are the key to achieve artificial general intelligence (AGI).
The current situation of machine learning systems can be summarized like this: supervised learning works but requires a lot of training data, unsupervised learning remains unpractical and RL applications have been mostly constrained to games. As a result, some of the top minds in the AI community have started turning their attention onto a new exciting area known as self-supervised learning. Conceptually, self-supervised learning focuses on building systems that convert an unsupervised problem into a supervised one and can learn with unlabeled data. This is analogous as babies develop a model of the world without being trained or interact physically too much with it but by simply observing. This week AI legends Yann LeCun and Yoshua Bengio described self-supervised learning as the future of AI.
Now let’s take a look at the core developments in AI research and technology this week.
AI Research:
More Efficiency in AI
OpenAI published an analysis demonstrating than algorithmic improvement has yield higher levels of efficiency compared to hardware advancements.
>Read more in this blog post from OpenAI
Understanding the Shape of Large Datasets
Researchers from Google published a fascinating paper proposing a graph-based method to understand patterns in large datasets.
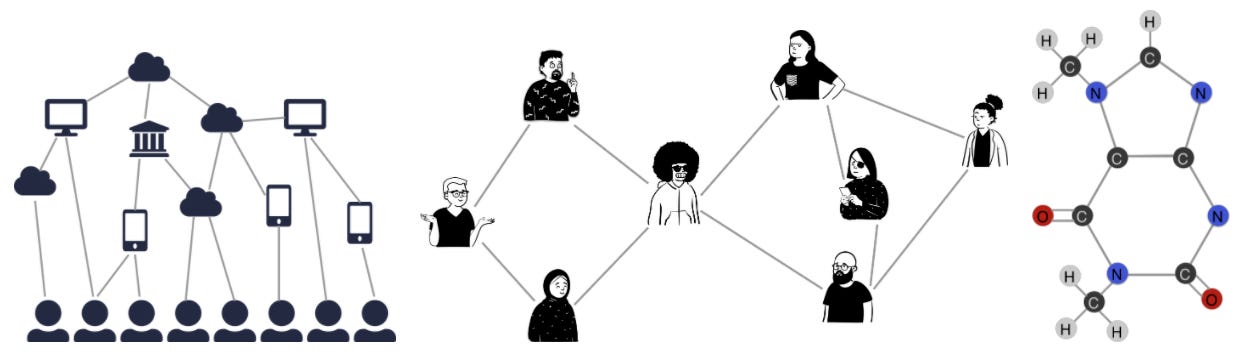
The image credit: Google AI
>Read more in this blog post from Google Research
How Alexa Knows When You Are Talking to Her
Amazon researchers published a paper a method based on semantic and syntactic features to improve the detection of device-directed speech.
>Read more in this blog post from Amazon Research
Cool AI Tech Releases:
Better TensorFlow-Spark Integration
Engineers from LinkedIn open-sourced Spark-TFRecord, a new library to leverage TensorFlow’s TFRecord as native Spark datasets.
>Read more in this blog post from the LinkedIn engineering team
StellarGraph
StellarGraph, a new framework focused on state-of-the-art graph machine learning, is now open source.
>Read more in this blog post from the StellarGraph team
AI in the Real World:
MinsDB
AI startup MinsDB raised $3 million to accelerate its platform that allows data scientists to rapidly train and deploy machine learning models.
>Read more in this coverage from VentureBeat
Microsoft CTO Book
Kevin Scott, CTO of Microsoft, has published a new book about how AI can reprogram the American Dream.
>Read more in this coverage from the Wall Street Journal
AI for Measuring Social Distancing
Andrew Ng’s AI startup Landing AI created a tool that uses image analysis to measure social distancing in the workplace.
>Read more in this coverage from MIT Technology Review
“This Week in AI” is a newsletter curated by industry insiders and the Invector Labs team, every week it brings you the latest developments in AI research and technology.
From July 14th the newsletter will change its name and format to develop ideas of systematic AI education.
To stay up-to-date and know more about TheSequence, please consider to ➡️