TheSequence Scope: The Biggest Roadblock for the Mainstream Adoption of Machine Learning
Initially published as 'This Week in AI', a newsletter about the AI news, and the latest developments in ML research and technology
From the Editor: The Biggest Roadblock for the Mainstream Adoption of Machine Learning
One word: data. Up to this day, we haven’t seen the complete potential of unsupervised models and supervised methods rule the machine learning space. Supervised training requires high quality labeled datasets and those are incredibly expensive to produce on an ongoing basis. These challenges have prevented even large organizations from adopting machine learning at scale and are a major roadblock for startups entering the space.
The labeled data hurtle has two-man solutions: Either we develop methods for producing labeled datasets more effectively or we develop methods that can learn with smaller datasets. In the first solution, areas such as generative models are showing some promise to generate high-quality training datasets. In terms of the latter, we have seen incredible advances in research in areas such as semi-supervised models, one-shot or zero-shot learning are improving very rapidly. While the adoption of these techniques remains in a very nascent stage, the problem that they are trying to solve remains the biggest challenge for the adoption of modern machine learning solutions.
Now let’s take a look at the core developments in AI research and technology this week.
AI Research:
Understanding Glass Using Neural Networks
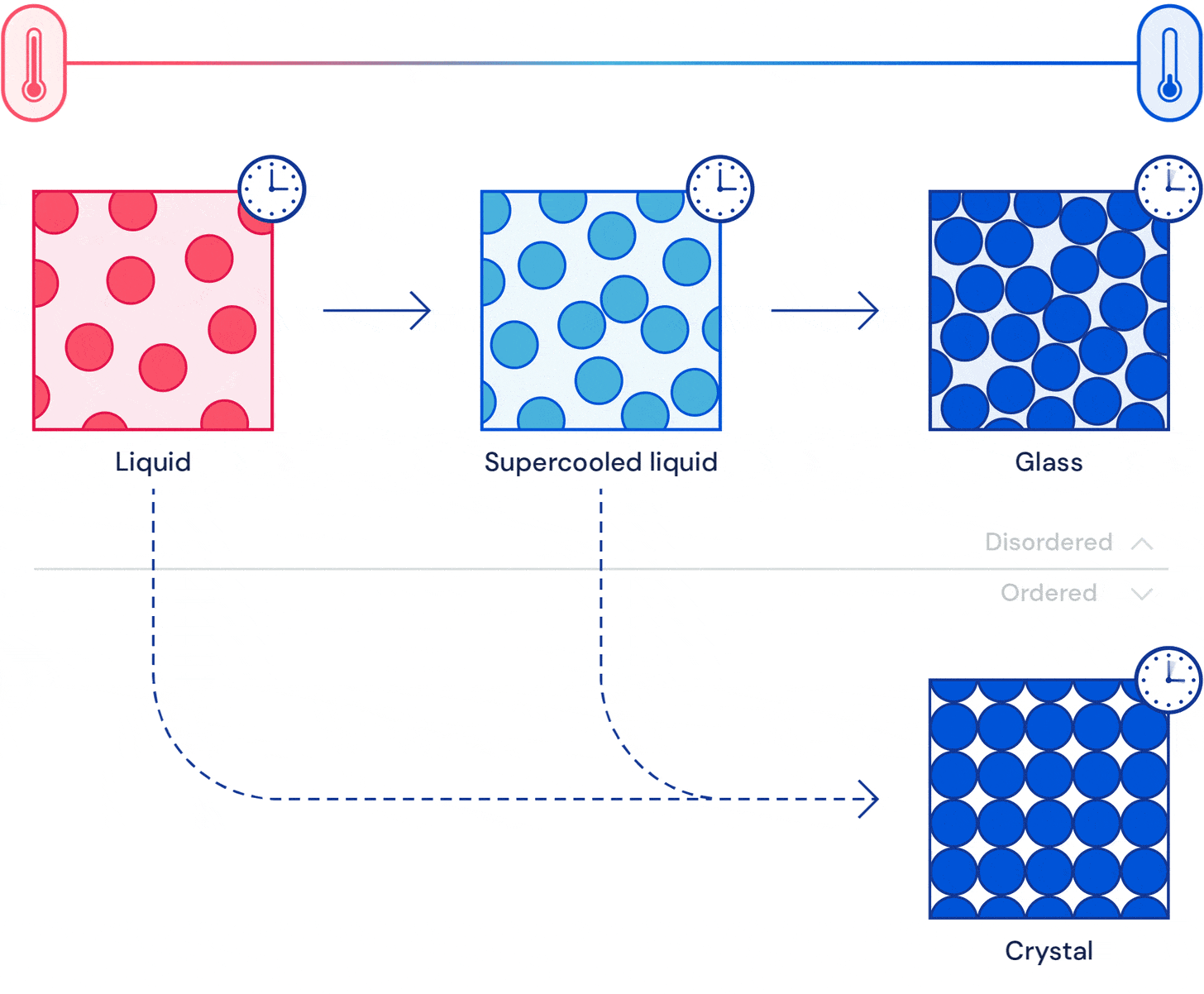
The image credit: DeepMind.com
DeepMind published a very intriguing paper proposing a method based on graph neural networks to understand the puzzling phenomenon of the glass transition.
>Read more in this blog post from the DeepMind team
Advancing Generative Networks
Microsoft Research published an insightful summary of three different projects in generative models that can facilitate the implementation of self-supervised learning methods.
>Read more in this blog post from Microsoft Research
Learning Tasks from Single Examples
Researchers from Amazon published a paper proposing a method that improves performance in meta-learning tasks without increasing the training data requirements.
>Read more in this blog post from Amazon Research
Cool AI Tech Releases:
TensorFlow QAT
The TensorFlow team released the Quantization Aware Training (QAT) API which allows the implementation of faster and smaller machine learning models.
>Read more in this blog post from the TensorFlow team
Sound Separation Dataset
Google open-sourced the Free Universal Sound Separation data set, intended to support the development of AI models that can separate distinct sounds from recording mixes.
>Read more in this blog post from the Google Open Source team
AI in the Real World:
$30M for AI Research
The US Department of Energy announced $30 million in funding for AI research projects.
>Read more in the official press release of the Department of Energy
Node AI
AI startup Node raised $6 million to help companies launch AI projects without requiring data science expertise.
>Read more in this coverage of VentureBeat
AI to Study the Oceans
We know very little about our oceans. AI methods are helping researchers to overcome some of the traditional challenges analyzing the super large datasets related to oceanic data.
>Read more in this coverage of the New York Times
“This Week in AI” is a newsletter curated by industry insiders and the Invector Labs team, every week it brings you the latest developments in AI research and technology.
From July 14th the newsletter will change its name and format to develop ideas of systematic AI education.
To stay up-to-date and know more about TheSequence, please consider to ➡️