In this issue:
we start a new series about MLOps;
we explore TFX, a TensorFlow-based architecture created by Google to manage machine learning models;
we overview MLflow, a platform for end-to-end ML lifecycle management.
💡 ML Concept of the Day: A New Series About MLOps Â
We couldn’t resist it any longer and decided to start a new series about machine learning operations (MLOps), considered one of the hottest topics in the ML world. The MLOps is becoming increasingly crowded with a large number of stacks ranging from technology powerhouses to innovative ML startups. As a result, it has become more difficult to keep visibility across the entire MLOps ecosystem.
One of the challenges to understanding MLOps is that the term itself is used very loosely in the ML community. In general, we should think about MLOps as an extension of DevOps methodologies but optimized for the lifecycle of ML applications. This definition makes perfect sense if we consider how fundamentally different the lifecycle of ML applications is comparing to traditional software programs. For starters, ML applications are composed of both models and data, and they include stages such as training or hyperparameter optimization that have no equivalence in traditional software applications.
Just like DevOps, MLOps looks to manage the different stages of the lifecycle of ML applications. More specifically, MLOps encompasses diverse areas such as data/model versioning, continuous integration, model monitoring, model testing, and many others. In no time, MLOps evolved from a set of best practices into a holistic approach to ML lifecycle management.
In this series, we plan to break down the building blocks of MLOps, explaining the concepts behind them, highlighting some of the top research papers and technology stacks in the MLOps space. Keep up!
🔎 ML Research You Should Know: TFX is Google’s Vision for Managing the Lifecycle of Machine Learning Models Â
In the paper TFX: A TensorFlow-Based Production-Scale Machine Learning Platform, researchers from Google outlined an architecture for the end-to-end continuous management of the lifecycle of machine learning models. Â
The objective: Illustrate the components of a TensorFlow-based architecture created by Google to manage ML models in mission-critical applications such as Google Play.  Â
Why is it so important: The TFX paper is widely recognized within the machine learning community as one of the first efforts to clearly outline the new architecture components needed to enable CICD in machine learning models. Â
Diving deeper: TensorFlow Extended (TFX) is the architecture proposed by Google Researchers for automating the lifecycle of TensorFlow models from experimentation to production. Fundamentally, the TFX framework is based on the following principles:Â Â
One machine learning platform for many learning tasks: TFX provides a consistent architecture for automating the lifecycle of different types of machine learning models. Â
Continuous training: TFX supports a pipeline for enabling continuous training workflows in TensorFlow models. Â
Easy-to-use configuration and tools: Configuration management is essential to automate the lifecycle of machine learning models. TFX provides a system that maps different components of a TensorFlow model to specific configuration settings. Â
Production-level reliability and scalability: TFX includes a series of building blocks to ensure that TensorFlow models built using the platform can operate at scale in production. Â
The TFX architecture includes several key components of the lifecycle of machine learning models. Â
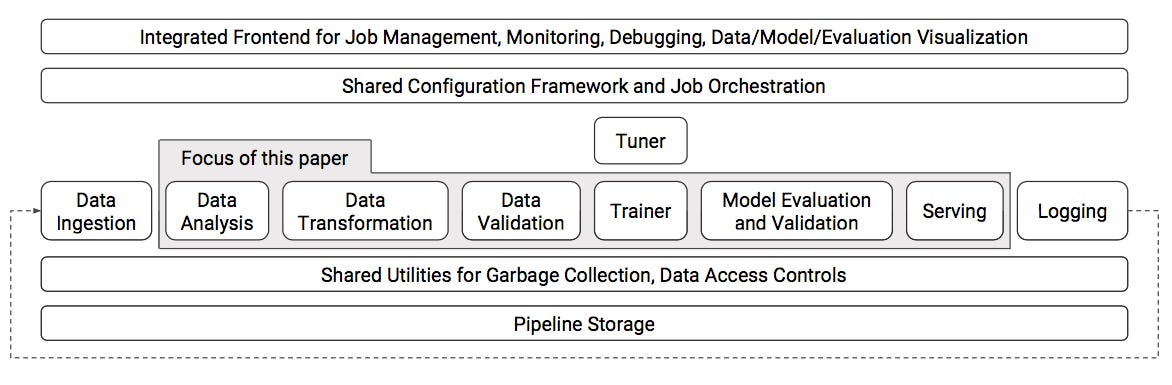
Data Analysis: TFX abstracts data analysis via a component that processes each dataset fed to the system and generates a set of descriptive statistics on the included features.Â
Data Transformation: This component of the TFX architecture implements a suite of data transformations to allow feature wrangling for model training and serving.Â
Data Validation: This component is responsible for validating the health of a given task after the data analysis face. Â
Model Training: This component is responsible for training TensorFlow models across as many possible use cases.Â
Model Evaluation: This component focuses on the evaluation of models prior to deploying them. Â
Model Serving: This component automates the deploying and serving of TensorFlow models.Â
TFX is powering mission-critical architectures within Google, such as the famous Google Play Store, which uses hundreds of TensorFlow models. An open-source implementation of TFX has been included in the TensorFlow project.    Â
🙌 Let’s connect
Follow us on Twitter. We share helpful information, events, educational materials, and informative threads that help you strengthen your knowledge about ML and AI.
🤖 ML Technology to Follow: MLflow Helps You Manage the Lifecycle of Machine Learning ModelsÂ
Why should I know about this: MLflow is one of the most popular platforms for end-to-end machine learning lifecycle management and is integrated with every major framework and platform in the market. MLflow enables many capabilities, such as model serving that enables the lifecycle management of machine learning models. Â
What is it: MLflow was incubated with big data pioneer DataBricks with the goal of simplifying the end-to-end lifecycle management of machine learning programs. Originally, MLflow drew inspiration from machine learning architectures such as  FBLearner Flow, TFX, and Michelangelo, developed by Facebook, Google, and Uber respectively, to manage their machine learning workflows. MLflow implements many of the principles of those architectures into an open-source framework that can be easily incorporated into any machine learning project. Â
From an architecture standpoint, MLflow is organized into four main components that abstract different stages of ML models’ lifecycle: Â
MLflow Tracking: This component is, essentially, an API for logging different elements of a machine learning model such as parameters, metrics, and versioning artifacts. Â
MLflow Projects: This component provides a standard format for structuring machine learning projects in a way that can ensure reproducible runs in platforms like Docker or Conda. Â
MLflow Models: This component is a packaging format for deploying machine learning models across different runtimes such as Apache Spark, Azure ML, and AWS SageMaker.Â
MLflow Model Registry: This component is a centralized model store, APIs, and UI to manage machine learning models' lifecycle. Â
In addition to those four building blocks, MLflow includes numerous subprojects that implement different capabilities in the lifecycle of ML models. One of those subprojects is MLflow Model Serving which adds model serving capabilities of the MLflow Registry. Using MLflow Model Serving, machine learning teams can easily operationalize multiple versions of machine learning models across different environments and expose APIs to third-party applications. Â
The components of the MLflow architecture can be combined to manage the lifecycle of any machine learning pipeline. One of the main advantages of MLflow is that it bridges the gap between data science research teams focused on experimentation and machine learning engineers tasked with deploying and scaling models in production. MLflow integrates with the popular machine learning frameworks such as TensorFlow, PyTorch, and MxNet, as well as machine learning runtimes like Azure ML, DataBricks, and AWS SageMarker. Â
How can I use it: MLflow is open source at https://github.com/mlflow/mlflow. Versions of the platform can also be used as part of platforms like Azure or AWS. Â