📝 Editorial
‘Data is the new oil’ is an over-marketed quote, but one that is certainly true when it comes to machine learning (ML). In an ML world dominated by supervised learning techniques, having access to high-quality labeled datasets is essential to advance ML research and practical implementations. However, labeled datasets are computationally expensive to produce and remain a privilege of large companies, which increases the gap between the “haves” and the “have nots” in the ML space.
Beyond the impact in the economics of the ML market, access to high-quality datasets is fundamental to advance research in different ML fields. Datasets such as ImageNet were kind of a Sputnik moment (we mean the first artificial satellite) in ML, sparking remarkable breakthroughs in computer vision. Different areas in ML require highly specialized training datasets, which are incredibly hard to produce. Think about what it takes to create datasets for very specific ML tasks such as fake news detection, bias analysis, adversarial robustness, question answering in Swedish, etc. and you can get an idea of the magnitude of the challenge faced by new data science teams trying to get access to those resources. Providing the right vehicles for large AI labs to open-source datasets and benchmarks is essential to address some of the fundamental challenges in modern ML solutions. Thankfully, we are making progress. Just this week, Google and Facebook open-sourced datasets for highly specialized areas, such as gender bias and image manipulation respectively, while the Linux Foundation provided an open-source license for this type of effort. Hopefully, we will continue to see similar efforts in the near future.
🔺🔻 TheSequence Scope – our Sunday edition with the industry’s development overview – is free. To receive high-quality content about the most relevant developments in the ML world every Tuesday and Thursday, please subscribe to TheSequence Edge 🔺🔻
🗓 Next week in TheSequence Edge:
Edge#101: we finalize the Reinforcement Learning series, discussing the Exploration-Exploitation Dilemma; review how Microsoft Research uses Bayesian exploration to address the exploration-exploitation dilemma in RL agents; explore TF-Agents, a modular RL Library for TensorFlow.
Edge#102 is about DeepMind’s fascinating paper that looks to redefine the Principal Component Analysis (PCA) algorithm as a competitive multi-agent game called EigenGame.
Now, let’s review the most important developments in the AI industry this week
🔎 ML Research
Self-Supervised Learning
Researchers from Salesforce published an insightful blog post about the evolution of self-supervised learning methods ->read more on Salesforce Research blog
Quantum ML
Google Research published a paper detailing the benefits and challenges of quantum machine learning ->read more on Google Research blog
LReasoner
Microsoft Research published a paper detailing LReasoner, a pretrained language model optimized for logical reasoning ->read more on Microsoft Research blog
🤖 Cool AI Tech Releases
Open Data License
The Linux Foundation announced the CDLA-Permissive-2.0 license to enable the sharing of open-source datasets ->read more on Linux Foundation blog
Gender Bias Dataset
Google Research released a dataset optimized for detecting gender bias in machine translation models ->read more on Google Research blog
Image Similarity Dataset
Facebook AI Research (FAIR) open-sourced the Image Similarity dataset designed for detecting fake and manipulated images in computer vision models ->read more on FAIR team blog
AWS BugBust
AWS announced the BugBust challenge, a global competition to fix one million bugs using AWS ML-powered developer tools such as CodeGuru ->read more on AWS team blog
💬 Useful tweet
Join our Twitter, we recommend things that you were looking for.

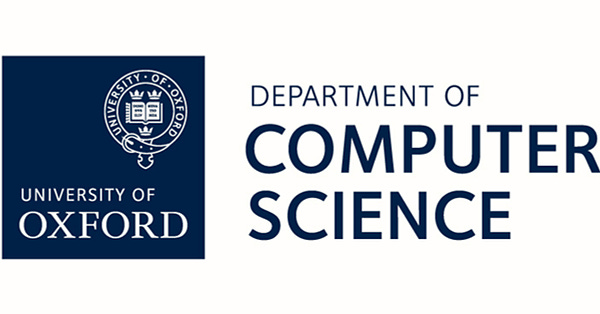
💸 Money in AI
We congratulate our partners, AI training platform Determined.AI, on the acquisition by Hewlett Packard Enterprise. They are hiring.
AI-based productivity tools maker Memory.ai raised $14 million in a round led by Melesio and Sanden. Hiring.
Data analytics software startup Incorta raised $120 million in a Series D round led by Prysm Capital. Many job openings.
Log management solution Graylog raised a $18 million growth equity round led by new investor Harbert Growth Partners and co-investor Piper Sandler Merchant Banking. Hiring in Engineering and Sales.
Feature store Rasgo raised a $25 million Series A funding round led by Insight Partners, with participation from Unusual Ventures.
NLP platform Primer raised a $110 million Series C round led by Lee Fixel's Addition. Hiring on many positions.
AI-powered platform for marketing optimization Tomi.ai raised $1 million in seed funding from Begin Capital and the Phystech Leadership Fund. FullStack JS developer and a data scientist are needed.
Compliance and security automation startup Drata raised a $25 million Series A round led by GGV Capital. Hiring.
AI-based platform for drug development and discovery Insilico Medicine raised $255 million in Series C financing led by Warburg Pincus. Interesting job openings.