🔮 The Future of Deep Learning According to Three Legends 🧙🏻♂️ 🧙🏻♂️ 🧙🏻♂️
📝 Editorial
Somebody, someday, should produce a movie about the history of deep learning. The story certainly doesn’t lack drama and has a few heroes. Among the many protagonists of that movie, there should be a special place for Yoshua Bengio, Geoffrey Hinton, and Yann LeCun. The three deep learning pioneers stayed faithful to the neural network paradigm even during the infamous AI winters where the majority of the AI community was looking for alternative architectures. Their efforts were rewarded not only with the current renaissance of deep learning but also with the Turing Award (which is considered to be the Nobel prize in computer science) in 2018. Last week, the three AI legends joined forces again to publish a paper that evaluates recent breakthroughs in deep learning methods and also challenges in the near future.
Titled “Deep Learning for AI”, the new paper addresses the tough question of the limits of neural networks and whether we need to resort back to more traditional symbolic representations (logic). Despite the progress in neural networks, many experts believe that there are intrinsic limitations of that type of architecture that require more traditional symbolic AI. This approach has been popularly called hybrid AI. Bengio, LeCun and Hinton categorically refuse this idea and they still believe that neural networks should evolve to master most aspects of human intelligence, including symbolic representations, common sense and logical inference. There are plenty of challenges that need to be addressed for neural networks to achieve those breakthroughs. Bengio, LeCun and Hinton believe that now we have a deep understanding of how to tackle those challenges. Deep Learning for AI is a very inspirational paper for new researchers journeying into the deep learning space. The next few decades of AI will show whether the theses outlined in this paper hold true or not.
🔺🔻TheSequence Scope – our Sunday edition with the industry’s development overview – is free. To receive high-quality content about the most relevant developments in the ML world every Tuesday and Thursday, please subscribe to TheSequence Edge 🔺🔻
🗓 Next week in TheSequence Edge:
Edge#105: Recap of '“What’s New in AI”
Edge#106: Recap of tech solutions that we’ve covered this year
Now, let’s review the most important developments in the AI industry this week
🔎 ML Research
Deep Learning for AI
Turing Awards winners Yoshua Bengio, Geoffrey Hinton, and Yann LeCun published a masterful paper exploring the recent breakthroughs and near term future of deep learning technologies ->read more in the original paper
Evaluating OpenAI Codex
Researchers from OpenAI published a paper evaluating the limitations of Codex, their GPT-based model for code generation that powers Github CoPilot ->read more in the original paper
Generative Models that can Extrapolate
Salesforce Research published a super interesting paper exploring generative models that can extrapolate knowledge without being fully trained in the subject ->read more on Salesforce Research blog
Text-to-Speech Synthesis with Appropriate Prosody
Amazon Research presented two papers describing techniques that help synthesize speech from text with the correct prosody->read more on Amazon Research blog
🤖 Cool AI Tech Releases
Rapid ML Experiments with CloudFlare
IBM announced the release of CloudFlare, an open-source framework to streamline machine learning tests ->read more on Microsoft blog
Elastic Training with XGBoost on Ray
Uber and Anyscale collaborated in the open-source release of XGBoost on Ray, a new framework for highly scalable distributed training ->read more on Uber engineering blog
Scrutinizing Machine Generated Text with SCARECROW
Researchers from the Allen Institute for AI published a paper detailing SCARECROW, a crowdsourced error annotation framework and toolset to scrutinize text produced by modern NLP models->read more on the project page
✏️ A Survey: Data Labeling for ML
Data labeling can be very confusing. Help us prepare an article about it by answering a few questions. The level of experience is not that relevant.
As a thank you, we will send you a cheat sheet with 40+ useful resources that help you understand and organize data labeling.
💬 Useful tweet
Nvidia unveiled Supercomputer Cambridge-1, a $100 million investment that promises to harness partnerships across the U.K. for breakthroughs with a “global impact.” Nvidia is making it available to external researchers in the U.K. health care industry.

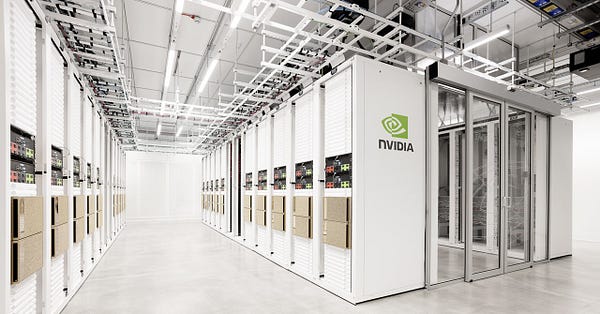
💸 Money in AI
ML-powered web conversion optimization platform Intellimize raised $30 million in Series B funding led by Cobalt Capital. Hiring.
Synthetic speech technology startup WellSaid Labs, a spinout from the Allen Institute for AI, raised a $10 million Series A led by FUSE. Hiring!
Data privacy startup Opaque raised a $9.5 million seed funding round led by Intel Capital. Hiring.
Database optimization startup Silk raised $55 million in a Series B funding round led by S Capital. Hiring.
AI-powered biometric solutions platform AnyVision raised $235 million in a Series C round co-led by SoftBank’s Vision Fund 2 and Eldridge.