💸🤷🏽♂️ Running AI Compute Infrastructures Without Breaking the Bank
📝 Editorial
Running an AI infrastructure is expensive. Not a month goes by in which I am not surprised by our GPU infrastructure bills and every other day I hear anecdotes of some of our data science experiments “running out of resources.”
Can we get smarter about this?
Data science is hard enough as it is, but now machine learning engineering teams need to be experts at resource allocation too. Even if it is unquestionable that platforms like AWS, Google Cloud and Azure have made a lot of progress adding GPU support in their machine learning platforms, the technologies for dynamic resource allocation, distribution or budget planning remain in their infancy.
Years ago technologies like virtual machines and containers revolutionized resource allocation in modern software architectures. Nobody really had to think about bare metal servers anymore and elastic resource allocation became the norm. However, AI infrastructures seem to have taken a step back. The current race of innovation in GPU technology and hardware acceleration has introduced new levels of complexity to manage resources. New, sexy AI hardware is really expensive and unpredictable to manage at scale.
Bottom line: modern AI needs better, more intelligent resource allocation and planning infrastructures. This is the mission of companies like Run:AI, which just this week completed a large $30 million Series B round. We need more efforts like this to build better AI infrastructures.
Now, let’s review the most important developments in the AI industry this week.
🔎 ML Research
News Ranking at Facebook
The Facebook engineering team published an insightful blog post about the machine learning architecture used to rank the news feed in the social network platform ->read more in this blog post from the Facebook engineering team
Improving Google Translate
Google Research published a paper describing the techniques used to reduce speech translation revisions in Google Translate ->read more on Google Research blog
Using Reinforcement Learning to Teach Robots to Manipulate Objects
Facebook Research published two papers detailing an impressive reinforcement learning model to teach robots how to manipulate objects using visual demonstrations ->read more on FAIR blog
“Liquid” Networks
New time-continuous recurrent neural network models (dubbed “liquid") that learn on the job, not just during its training phase, were introduced by researchers from MIT, IST Austria and TU Wien ->read more in the original paper or on MIT blog
🤖 Cool AI Tech Releases
Dialogflow CX
Google released the general availability version of Dialogflow CX, a service to build virtual conversational agents ->read more on Google AI blog
Model Anonymization Tool
IBM released a framework that uses differential privacy to anonymize machine learning models ->read more on IBM Research blog
Smart-Arg
LinkedIn open-sourced Smart Argument Suite, a framework to connect Python jobs in machine learning pipelines ->read more in this blog post
💬 Useful Tweet
We strongly recommend reading this AI Ethics Report (Jan 2021) by Montreal AI Ethics Institute with chapters on Algorithmic Injustice, Discrimination, Ethical AI, Labor Impacts, Misinformation, Privacy, Risk & Security, and Social Media; and following them on Twitter.

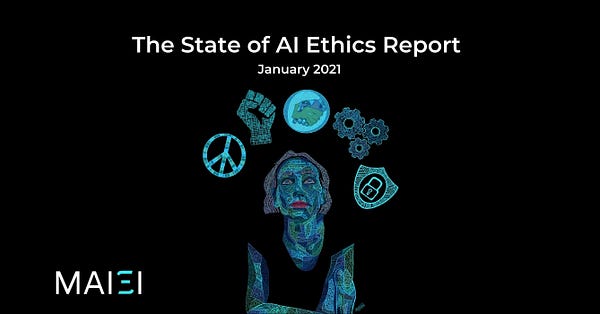
Interested in
job listing or sponsoring TheSequence
? Let us know by replying to this email.
💸 Money in AI
ML and AI startups:
Deep learning orchestration platform Run:AI raised $30 million in a Series B round. The startup addresses the challenge of limited ability to allocate and control expensive compute resources to achieve optimal speed and utilization, by building a compute-management platform for virtualizing, orchestrating and accelerating AI workloads.
The vector database for ML Pinecone Systems raised $10 million in funding. It provides the infrastructure for ML applications that need to search and rank results based on similarity. Pinecone’s database supports deployments of personalization, semantic text search, image retrieval, data fusion, deduplication, recommendation, anomaly detection, and other real-time applications, all with a simple API and zero maintenance.
Annotation platform Kili Technology raised $7 million. The startup creates tools that help enterprises annotate raw data such as video, drone aerial images, contracts, and emails more efficiently. There is strong competition among annotation platforms. Last year, we’ve mentioned that Dataloop raised $16 million. SuperAnnotate raised $3 million, and IBM also released annotation tools that tap AI to label images.
AI and ML implementation:
Biotech company Ukko raised $40 million in a Series B. The startup applies ML technologies in immunology, computational biology, and protein engineering to develop new approaches to treating food sensitivities. They use algorithms to predict such things as what in the peanut protein is triggering the allergic attack by the immune system.
Cloud data analytics company Phocas raised $34 million. Simplifying data management through analytics and visualization tools, they use AI to extract value from data and enable businesses to run operations without cross-referencing spreadsheets.
“Knowledge-as-a-service” platform Lynk raised $24 million. Using varied AI tools, Lynk connects investors and companies to vetted global experts from companies like Airbnb, Pfizer, Wells Fargo etc. They also have functions that allow customers to harness more from that knowledge, such as team collaboration, workflow management and automatic transcription.
Deep learning and 3D modeling platform for industrial engineering Physna raised $20 million. Using proprietary algorithms and advanced geometric deep learning technology, Physna codifies 3D models into detailed data that is understandable by software applications.
Supplier intelligence startup Tealbook raised $14.4 million in a funding round. Tealbook leverages ML to bring its customers always-updated supplier data, while unifying and synchronizing it. It gathers fragmented information from disparate, hidden sources across the web, then blends that information into a complete data picture.
Supply Chain Intelligence company Verusen raised $8 million in a Series A round. Their technology enables an AI-based self-cleansing and harmonization of disparate supply chain data, while allowing real-time field knowledge input into its machine learning capabilities.