☝️⚖️ ML Fairness is Everybody’s Problem
📝 Editorial
We typically associate fairness issues in machine learning (ML) models with large consumer tech startups like Facebook, Apple and Twitter. It seems easy enough to point the finger at biased decisions by ad-selection algorithms in Facebook or Apple’s credit evaluation models. While these examples are certainly visible and impactful, we should also understand that fairness is one of the hardest problems of modern ML. After all, let’s keep in mind that we haven’t quite figured out fairness in modern socio-economic models and we are still living in a world where bias, discrimination and dictatorial regimes are fairly prominent. The socio-economic fabric of modern societies has been built over centuries of unfairness and discrimination, so it’s not like modern AI systems have the best teachers to learn from 😉 But let’s not get carried away because, after all, this newsletter is about ML technology and not philosophy.
To put some context to the monumental challenge that companies like Apple or Facebook face when it comes to ML fairness, let’s try to think about what we are doing to introduce fairness in our small-scale ML solutions. I am going to guess that very little 😉 And this is not surprising because building ML fairness constructs is brutally hard. Our best bet seems to be ML monitoring platforms like Tensor Board, Fiddler or Arthur AI, which are recently incorporating fairness indicators as part of the monitoring of ML models. Just this week, Facebook AI Research published a very comprehensive analysis of the research behind the fairness tools they are incorporating into ML models. The research is remarkable but so are the challenges. Certainly, fairness mechanisms in ML models are not an exclusive problem of large consumer tech platforms, but rather one of the building blocks of any modern ML solutions.
🔺🔻TheSequence Scope – our Sunday edition with the industry’s development overview – is free. To receive high-quality content about the most relevant developments in the ML world every Tuesday and Thursday, please subscribe to TheSequence Edge 🔺🔻
🗓 Next week in TheSequence Edge:
Edge#77: the concept of Feature Store; the story of how Uber Michelangelo started the Feature Store movement; the exploration of the feature store market.
Edge#78: Feast – an open-source, lightweight feature store.
Now, let’s review the most important developments in the AI industry this week
🔎 ML Research
Fairness Flow
Facebook AI Research( FAIR) published a very insightful blog post describing the techniques used to build Fairness Flow, a tool to evaluate the fairness of machine learning models ->read more on FAIR blog
More AI Behind Alexa
Amazon AI Labs published a great blog post summarizing some of the recent AI breakthroughs incorporated into the Alexa digital assistant ->read more on Amazon AI Labs blog
AI that Names Weird Colors
Facebook AI Research (FAIR) published a research paper about two neural networks that organically developed a convention to name strange colors very similar to the way humans do ->read more on FAIR blog
🤖 Cool AI Tech Releases
Dataiku 9
Dataiku unveiled the new version of its data science platform with a special focus on capabilities for non-technical users ->read more on Dataiku blog
💬 Futuristic Tweet
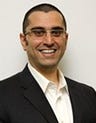
💸 Money in AI
AI-powered fintech software company HighRadius raised $300 million in a Series C funding round. It leverages AI-based autonomous systems to help companies automate accounts receivable and treasury processes. It also enables teams to leverage ML to predict future outcomes and automate routine labor-intensive tasks.
AI-powered mentoring startup Cresta, raised $50 million in a Series B round of funding. Its AI learns from the best performers of client’s customer service and then provides real-time AI mentoring, best practices, and feedback across all customer communications.
Contextual analytics startup Windfall raised $21 million in venture capital. It provides a 360-degree view of a client’s database to accelerate workflows and drive better performance. One of the Windfall business’ focuses is nonprofits. The platform helps estimate potential donors’ net worth at the household level, leveraging data and machine learning to make projections.
Auditoria raised $15.5 million in Series A funding. By leveraging NLP, AI, and ML, Auditoria's platform automates back-office business processes, including both routine tasks and complex functions, such as predictive analytical forecasting.
Conversational AI startup Uniphore raised $140 million in a Series D round of funding. Its solutions transform customer service by providing an automation platform where digital agents take over transactional conversations from humans, coach agents during calls, and accurately predict language, emotion and intent.
Account engagement platform 6sense raised $125 million at a valuation of $2.1 billion, a Series D. Its platform uses AI and big data architecture for sales and marketing functions such as identifying and engaging target accounts.
AI-powered virtual recruiting service Celential.ai raised $9.5 million in Series A funding. Its Virtual Recruiter service uses precision matching and 360-degree mutual-fit assessment, simulating human experts, to match candidates to job openings from its graph of over three million software engineers in the U.S. and Canada.
Decentralized data cloud (DDC) platform Cere Network raised $5 million. Its ambition is to compete with data cloud leader Snowflake by leveraging blockchain technology and more efficient AI/ML tools.