🛠 Introducing the Real World ML Section
We keep you updated with the most important things that happen in the ML world
📝 Editorial
Building machine learning (ML) solutions at scale remains an unexplored territory for most companies. Most data science teams have solid ideas of managing the lifecycle of a handful of ML models but how does an ML infrastructure for hundreds of thousands of models look like? Even though the MLOps space has been growing at a rapid pace, the architectures and best practices for applying those stacks at scale are being learned by trial and error. In the current ML market, some of the most advanced ML infrastructures are being built by large technology companies such as Facebook, Google, Uber, LinkedIn, Netflix and others. Analyzing those architectures is one of the most efficient ways to understand the potential challenges and solutions of large-scale ML architectures.
With this edition of TheSequence Scope, we have added a small section titled Real World ML. The objective of this section is to highlight new, documented best practices adopted in some of the largest ML infrastructures in the world. We think that systematically studying the ML architectures and techniques implemented by large technology companies is one of the best sources of inspirations you can find in the ML world. We hope the Real World ML section will help evangelize some of these ideas. For this week, we’ve included some new details about ML use cases at Uber and LinkedIn.
Happy Reading!
🔺🔻TheSequence Scope – our Sunday edition with the industry’s development overview – is free. To receive high-quality content about the most relevant developments in the ML world every Tuesday and Thursday, please subscribe to TheSequence Edge 🔺🔻
🗓 Next week in TheSequence Edge:
Edge#109: The start of the Transformers series (exciting!)
Edge#110: Overview of Pachyderm, a platform to streamline machine learning experimentation
Now, let’s review the most important developments in the AI industry this week
🛠 Real World ML
Orders Near You
The Uber engineering team published a detailed blog post about the implementation of the orders near you, a feature in the Uber Eats app ->read more on Uber Engineering blog
Large Scale Data Analytics at LinkedIn
The LinkedIn engineering team published a blog post detailing the architecture of their big data pipelines to power analytics workloads ->read more on LinkedIn Engineering blog
🔎 ML Research
BlenderBot 2.0
Facebook AI Research published a paper detailing the second version of its BlenderBot chatbot that incorporates long-term memory and internet knowledge capabilities ->read more on FAIR blog
Feature Learning with Super Wide Neural Networks
Microsoft Research published a paper proposing a technique capable of feature learning in infinitely scalable deep learning models ->read more on Microsoft Research blog
Vision-Language Contrastive Learning
Salesforce Research published a paper detailing ALign BEfore Fuse (ALBEF), a model that uses contrastive learning to achieve state-of-the-art performance in different language-vision tasks ->read more on Salesforce Research blog
🤖 Cool AI Tech Releases
TensoRT8
NVIDIA open-sourced the new release of its popular TensorRT framework designed for high speed, large scale inference jobs ->read more on NVIDIA Developer blog
TonY Goes to the Linux AI Foundation
LinkedIn’s TonY is a framework designed to enable the training of deep learning models in a Hadoop infrastructure, it just joined the Linux AI Foundation as an incubation project ->read more on LinkedIn Engineering blog
Facebook FSDP
Facebook open-sourced Fully Sharded Data Parallel (FSDP), a framework for large scale training with fewer GPU resources ->read more on Facebook Engineering blog
🗯 Useful tweet
NetHack Challenge, which we’ve covered in Edge#100, announced a new track

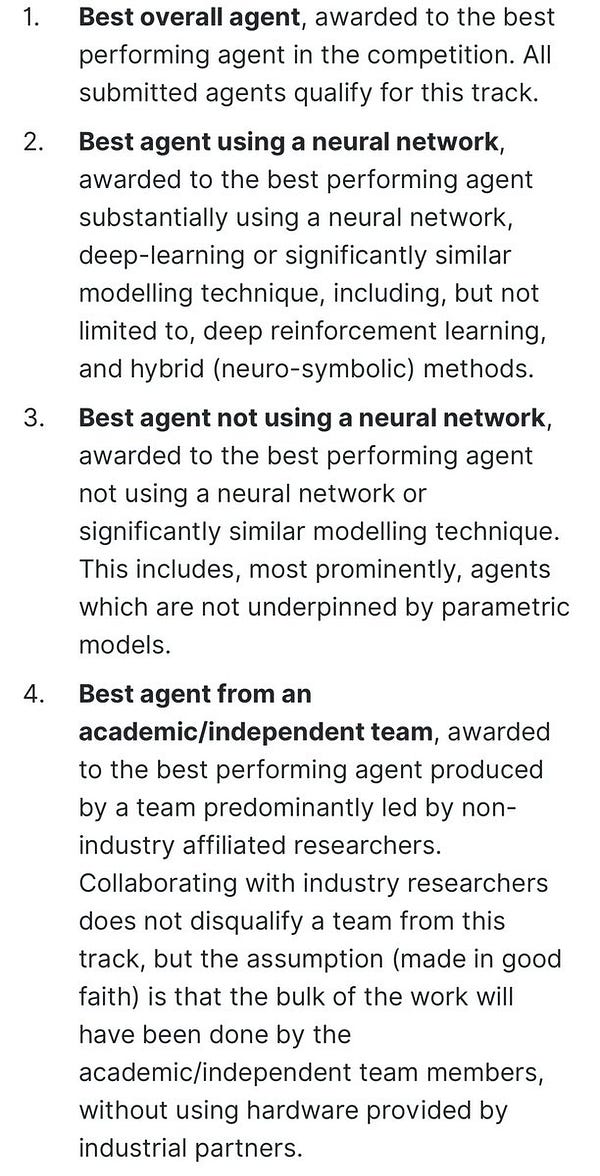
💸 Money in AI
For devs and engineers:
High-performance AI chips startup Untether AI raised $125 million from Tracker Capital Management and Intel Capital. Many positions in hardware and software.
Cube Dev, a core developer behind the open-source “analytical API platform” Cube.js, raised $15.5 million in a Series A round of funding led by Decibel. Hiring in the US or remote.
Graph analytics and ML startup Lucata raised $11.9 million in Series B funding, bringing its total raised to nearly $30 million. Three engineering positions in the US.
AI implementation:
Bayesian-based risk prediction engine Safe Security raised $33 million in a strategic investment led by BT Group. Hiring for many positions in Delhi/Mumbai.
AI-driven threat identification DNSFilter raised a $30 million Series A funding round led by investment firm Insight Partners. Looking for fully remote test engineer.
ML-enhanced SaaS platform for brand strategy insight BlueOcean raised a $15 million Series A round led by Insight Partners. Two job offerings in engineering.
Computer vision-based video editor startup VOCHI raised an additional $2.4 million in a “late-seed” round led by Genesis Investments.