🙈🙉🙊 GPT-3 and Large Language Models can Get Out of Control
📝 Editorial
Arnold Schwarzenegger really ruined AI for us. When people think about dangerous uses of AI, images of killing robots, à la Terminator, pop into their heads. While the Terminator version of AI is great for news headlines, it's completely outside the capabilities of today’s AI technologies. Instead of fantasizing about killer robots, we should turn our attention to other areas of AI that, if used inappropriately, can become extremely toxic and dangerous. From those areas, the most prominent example could be language pre-trained models, such as OpenAI’s GPT-3 or Google’s Switch Transformer.
Just to clarify, I am an optimist by nature and I think language pre-trained models and transformer architectures are the biggest milestone in the last five years of AI.
However, it is undeniable that the capabilities of language pre-trained models can get out of control very quickly. Applying those models to malicious scenarios such as deep fake generation, disinformation or bias doesn’t take a lot of technical effort. While today large language pre-trained models are under the control of somewhat reputable companies like OpenAI, Microsoft and Google, it is only a matter of months before those capabilities are recreated by other entities. Widespread adoption of large language pre-trained models can result in chaos. Just this week, researchers from OpenAI and Stanford University published a very insightful paper raising awareness about these risks.
Language pre-trained models are amazing, but they are also going to push the ethical boundaries of the current generation of AI companies.
Now, let’s review the most important developments in the AI industry this week
🔎 ML Research
Capabilities and Limitations of GPT-3 Like Models
Researchers from OpenAI and Stanford University published a summary of discussions exploring the capabilities and tangible limitations of large language models like GPT-3 or Google’s Switch Transformer ->read more in the original research paper
Multilingual Models Based Trained on a Single Language
IBM Research published a paper proposing a technique that enables machine learning models to master tasks in several languages, even if they have only been trained in a single one ->read more on IBM Research blog
Protecting Classifiers Against Adversarial Attacks
Microsoft Research published a paper introducing a technique called denoised smoothing, which can improve the robustness of classifiers without the need of retraining ->read more on Microsoft Research blog
🤖 Cool AI Tech Releases
TensorFlow 3D
Google Research open-sourced TensorFlow 3D, a library for machine learning models in 3D environments ->read more on Google Research blog
Apache Superset
Airbnb published an insightful blog post detailing their practices to scale Apache Superset’s self-service BI platform ->read more on Airbnb’s engineering blog
LinkedIn Fairness Indicators
LinkedIn’s engineering team published a detailed blog post explaining the use of its fairness indicator toolkit in large scale machine learning models ->read more in this blog post from the LinkedIn engineering team
💬 Useful Tweet
Every week we share the best lectures, courses, and books that you can get for free

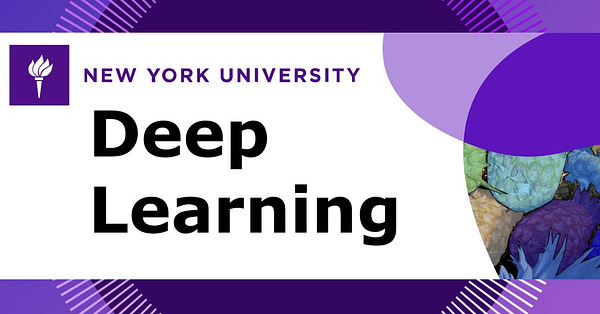
💸 Money in AI
For ML & AI
Data annotation and labeling platform Labelbox has raised $40 million (kudos to the team! 👏). Labelbox is the leading training data platform for enterprise ML applications, they created a complete workflow to organize and manage data, people and processes more effectively.
Data reliability startup Monte Carlo raised $25 million. Monte Carlo uses machine learning to infer and learn what a client’s data looks like, proactively identify data downtime, assess its impact, and notify those who need to know.
A semiconductor start-up NeuReality raised $8 million in a seed round, coming out of stealth mode. NeuReality’s purpose-built AI computing system architecture is designed specifically for the increasing complexity and scale of AI inference applications, enabling the scale of real-life AI applications.
AI implementation
Cybersecurity optimization solutions CYE raised over $100 million in a financing round. Hyver, CYE's flagship product, uses advanced algorithms and graph modeling to conduct a comprehensive cybersecurity assessment, covering the entire organization, as well as third-party vendors.
Biotech company Immunai raised $60 million in a Series A round. The startup combines single-cell genomics with ML algorithms to enable high-resolution profiling of the immune system. AI maps data to hundreds of cell types and states, extracting insights that lead to the discovery and development of more effective and targeted immunotherapies.
AI-guided antibody design platform BigHat Biosciences raised $19 million in a Series A round. BigHat’s wet lab is actively producing and characterizing antibodies at scale. The cloud-based AI/ML platform improves designs each round by combining active learning with assay modeling of critical biophysical properties, leading to the highest quality sequences.
Surgical platform developer Theator raised $15.5 million in a Series A round. They are doing pretty cool things: using AI and computer vision, the platform extracts and annotates every key moment from real-world procedures, giving the surgeons an opportunity to gain deep scientific insight into their own performances and those of surgeons worldwide.
AI-powered workplace safety startup Intenseye raised a $4 million seed round. Using computer vision and connected cameras, Intenseye empowers EHS teams to operationalize health and safety best practices across their facilities.
Language understanding, no-code platform Lang.ai, raised a $2 million seed funding round. The platform enables the next generation of NLP products and applications to scale processes that deal with text, from surveys to call centers to chatbot building.