🐟 👀 Edge#178: Supporting the Fight Against Illegal Fishing with AI
This Thursday, we decided to showcase a real-life implementation of artificial intelligence models and machine learning tools. Our goal is to keep you up to date with new developments in AI and real cases to complement the concepts we debate in other editions of our newsletter.
💥 What’s New in AI: Supporting the Fight Against Illegal Fishing
Estimates from the UN Food and Agriculture Organization (FAO) suggest that illegal fishing is responsible each year for the loss of 11–26 million tons of fish worth an estimated $10–23 billion. A global issue with almost 90 percent of fish stocks now fully exploited or overfished, any illegal, unreported and unregulated (IUU) fishing is a significant risk to the ocean’s vitality.
A collaboration of researchers from Skylight, AllenMLI (Machine Learning Impact), and PRIOR teams at the Allen Institute for AI (AI2) have developed AI models to assist in the fight against illegal fishing. AI2’s approach to integrating AI algorithms in the Skylight platform provides authorities insights needed to clamp down the illegal fishing crisis.
Skylight’s approach to Maritime Challenges
The metaphor “a needle in a haystack” aptly applies to the enormity of the task facing those seeking to combat illegal fishing in the vast waters of the world’s oceans. The challenge is immense, but so too is the potential payoff.
Seeing through the dark with computer vision
Governments, organizations, and the international community are looking to emerging technologies to identify illegal fishing activity. A key challenge to the puzzle is detecting vessels that are not transmitting their location, known as “dark” vessels, and may be fishing illegally. For this issue, AI2 researchers developed a computer vision model to detect vessels that may be trying to evade detection.
Synthetic Aperture Radar (SAR) satellite imagery offers the ability to “see” through clouds and at night. Low resolution, publicly available SAR is suitable for detecting metal objects, such as vessels, but is difficult for humans to process. This is especially true at scale where human resources are limited and the area to monitor is vast, like a country’s exclusive economic zone, which is typically about the width of California. This is where computer vision comes in. Computer vision offers the ability to quickly scan thousands of square miles in minutes to detect vessels. Correlating this data to known vessels transmitting their location using vessel tracking technologies quickly highlights “dark” vessels that may be seeking to evade detection.
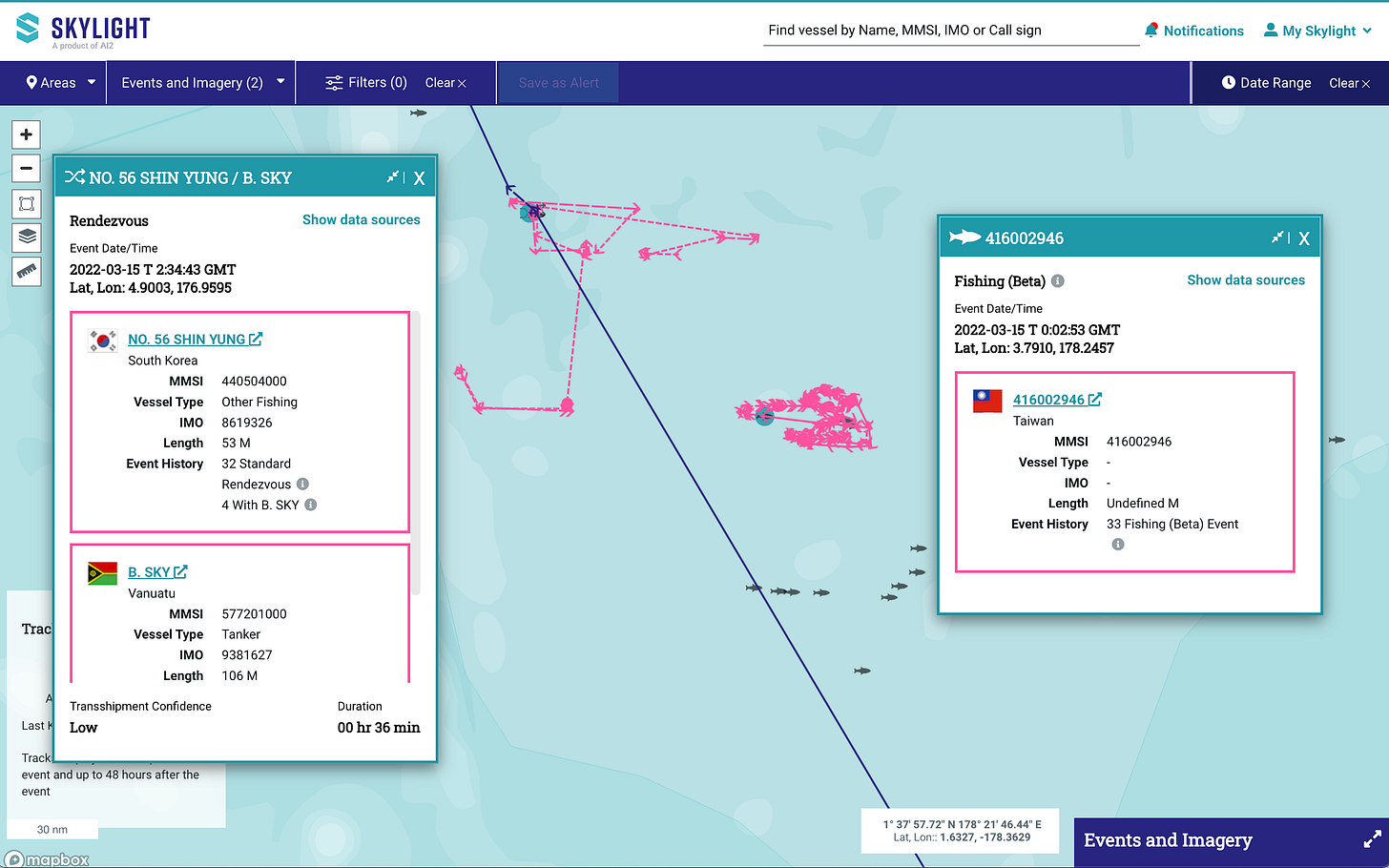
Challenges
Detecting vessels with computer vision comes with its own challenges. One of the biggest is overcoming the “noise” inherent to the natural world. This noise includes waves as well as rocks and other small land masses that can resemble vessels. The annotation of training data for the AI models is also a huge challenge due to waves, rocks, reefs, buoys, and the sheer volume and scale of the imagery. Finally, the delivery of this data has improved in recent years, down to mere hours, but the delay remains a challenge to effectively operationalize the information for interdicting suspicious vessels.
Despite these challenges, the detection of vessels, as well as the confidence to know areas where vessels are not, allows authorities to save resources by narrowing the scope of their patrol areas.
A Machine Learning approach to detecting fishing activities
Anomaly detection is a process of identifying items or events in data that do not conform to the expected pattern. For example, fraudulent credit card transactions can be detected by identifying patterns that differ from normal spending behavior. For the Skylight team and its partners, the goal is to identify the precise time and location of fishing or other related behaviors.
At AI2, time-series machine learning analysis is used to identify fishing by analyzing data from vessel tracking systems like the automatic identification system (AIS). Beyond fishing, this approach offers the ability to detect potential meetings with another vessel to “transship” cargo where only one vessel is transmitting AIS while another vessel may be “dark” and not transmitting AIS. Such events where fishing vessels meet fish carriers are a significant challenge to transparency in the global seafood supply chain.
Deterministic algorithms complement the machine learning models to detect other types of vessel behavior of interest to analysts, such as a rendezvous between two or more vessels transmitting AIS. While many of these events are legal, they can contribute to illegal fishing practices or even narcotics and human smuggling.
With hundreds of thousands of vessels and boats plying the world’s oceans, these powerful tools can identify where and when a vessel is engaging in potentially illegal or non-compliant behaviors for both on the water operations, compliance checks at port, and historical research.
In total, Skylight currently detects five kinds of events:
● Rendezvous Events
● Fishing Events
● Entry Events
● Speed Range Events
● Vessel Detection in SAR Imagery
Conclusion
AI offers a breakthrough in helping governments and organizations work to clamp down on illegal fishing, enabling them to efficiently monitor and enforce regulations against bad actors. From identifying fishing in restricted areas to seeing “dark” vessels at night and in any kind of weather, AI-assisted maritime monitoring is quickly becoming an important step in preserving the health of our ocean.
As more data becomes available and satellite imagery and processes continue to accelerate, the quality and time to deliver these insights will continue to close the gap on bad actors’ ability to hide.