🎈 Are Feature Stores the Next Bubble in AI?
Weekly newsletter that discusses impactful ML research papers, cool tech releases, the money in AI, and real-life implementations
📝 Editorial
Feature stores (feature store enables a centralized catalog of features in machine learning pipelines, covered in Edge#10) have been steadily becoming one of the key building blocks in modern machine learning architectures. Arguably, the concept of a feature store can be traced back to the original Uber’s Michelangelo architecture, having gone all the way from being an imperceptible capability to a booming standalone market in the machine learning space. These days, there is a growing number of venture-backed startups that are building feature store capabilities for machine learning solutions. Just this week, machine learning startup Molecula raised a solid $17.6 million round for their feature store technology.
With that disproportionate growth, you can’t help but wonder if there is a little bubble in the feature store market. I am a big fan of the concept of feature stores as well as a user of the technology, but I believe there are a few questions that are worth considering when analyzing this emerging capability.
Are feature stores a standalone product or a capability of broader platforms?
Can feature stores develop as a standalone market?
Is a potential feature store market big enough for so many companies?
How many feature store platforms do we really need?
I certainly have my own thesis about the feature store space, which I find incredibly fascinating. Let’s keep in mind that platforms like AWS have already entered the feature store space with the release of the AWS Feature Store. We should expect similar moves from Microsoft and Google soon. Those market developments place an additional relevance on the aforementioned questions. For now, the feature store space is enjoying a tremendous level of innovation and certainly has the attention of the venture capital community. However, is it sustainable?
🔺🔻TheSequence Scope – our Sunday edition with the industry’s development overview – is free. To receive high-quality content about the most relevant developments in the ML world every Tuesday and Thursday, please subscribe to TheSequence Edge 🔺🔻
🗓 Next week in TheSequence Edge:
Edge#55: the concept of DeepAR; overview of Amazon Research about multi-dimensional time-series forecasting; and sktime – a unified time-series framework for Scikit-Learn.
Edge#56: deep dive into DeepMind’s MuZero, a deep learning model that mastered Go, chess, Shogi and Atari without knowing the rules.
Now, let’s review the most important developments in the AI industry this week.
🔎 ML Research
Google Research 2020 Summary
Google Research published a very comprehensive summary of their areas of focus and contributions in 2020 ->read more on Google Research blog
Better Vision-Language Models
Microsoft Research published a paper proposing VinVL, a new multimodal vision-language model that relates visual and textual concepts ->read more on Microsoft Research blog
A Financial News Bot
IBM Research published a paper proposing a bot that can make sense of financial time series information and news ->read more on IBM Research blog
Recognizing Human Poses
Google Research published a paper discussing an algorithm that can recognize similarity in human body poses across different camera views ->read more on Google Research blog
🤖 Cool AI Tech Releases
MLMD
The TensorFlow team open-sourced machine learning metadata (MLMD), a TensorFlow library to track the complete lineage of machine learning models ->read more on TensorFlow blog
Robustness Gym
Salesforce Research open-sourced Robustness Gym, an environment to evaluate the robustness of natural language models ->read more in the project’s Github repository
💬 Useful Tweet

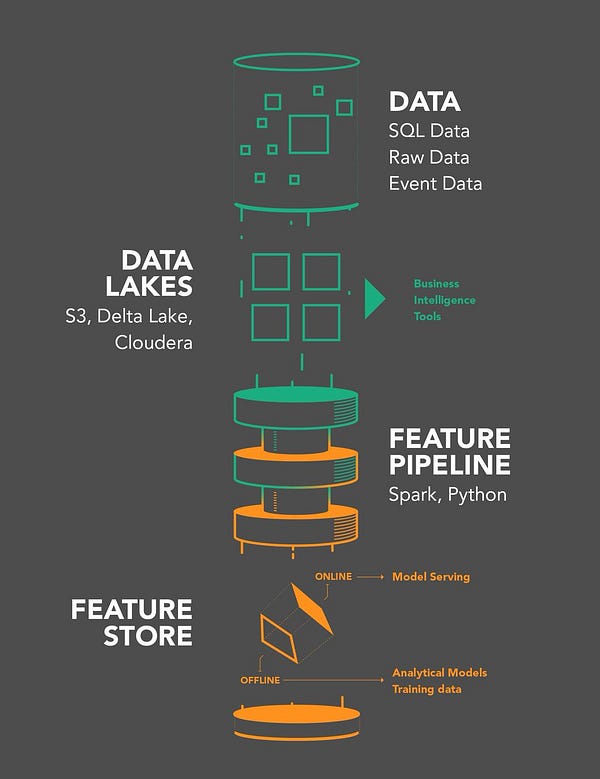
💸 Money in AI
Automation with AI:
🦄 Continuous integration and delivery platform for DevOps Harness raised $115 million at a $1.7 billion valuation. The company leverages machine learning and AI techniques to automate software deployments, monitor their quality, and enable rollbacks when flaws are discovered.
🦄 Intelligent automation platform Workato raised $110 million at a post-money valuation of $1.7 billion. Using AI, Workato lets companies integrate a range of data and apps to automate backend and front-end business workflows, complementing it with chatbot capabilities.
Content management startup M-Files raised $80 million in new funding. Its proprietor AI tools aim to automate the process of organizing and tracking internal documents and data for enterprises.
Personification with AI:
Personalized learning startup Sana Labs raised $18 million in a Series A round. The startup uses ML to personalize training courses for professionals. AI tools analyze learning behavior, identify patterns in learners data and provide insights in real-time.
Behavioral health startup NeuroFlow raised $20 million in a Series B round. Using AI — including natural language processing and behavioral analytics — the company aims to identify and treat consumers with depression, anxiety and other behavioral health conditions. AI also helps to automate processes like sending motivational emails, reminders etc.
AI-powered virtual assistant Dina raised $7 million. Dina offers AI-powered tools that analyze patient data to identify and recommend evidence-based interventions.
Predictions with AI:
Predictive analytics for healthcare startup Lumiata raised $14 million in a Series B round. Lumiata products are built from more than 120 million patient records that are ingested, cleansed, and organized into a unified record and enriched with annotations that make it ready for machine learning. Such an AI-powered approach allows the company to create superior cost and risk predictions.
AI extension for human memory startup Human AI raised $3.2 million in a seed round. Through a convergence of neuroscience, NLP and blockchain, they aim to create a digital version of each person's memory with a personal AI to retain and recall the memories.
For easier ML and AI implementation:
Feature store startup Molecula raised $17.6 million in Series A funding. The feature stores allow the automating of the preparation of data for machine-scale analytics and AI. Molecula offers data engineers and data scientists a cloud-agnostic, compute-ready data layer that is ready for high performance, high-concurrency, advanced analytics and AI.
End-to-end computer vision platform Roboflow raised a $2.1 million seed round. The startup helps companies that don’t have the in-house expertise to set up the infrastructure for building computer vision models and putting them into production.
Interested in sponsoring TheSequence? Let us know by replying to this email.
TheSequence is a summary of groundbreaking ML research papers, engaging explanations of ML concepts, exploration of new ML frameworks, and platforms. It also keeps you up to date with the news, trends, and technology developments in the AI field.
5 minutes of your time, 3 times a week– you will steadily become knowledgeable about everything happening in the AI space.
IT would be great if this article would provide a brief introduction to the concept of feature store, or even a definition.