🕹 A Massive Leap in ML for Gaming
📝 Editorial
Gaming has been at the center of the ML renaissance for the last few years. In many ways, the deep learning race was kicked off by AlphaGo performance again Go’s legend Le Sedol. However, most of the advancements in ML for gaming have been specialized in either perfect or imperfect information games but never both at the same time. Models that mastered chess and Go struggle with imperfect games such as Poker. Even models like AlphaZero, which learned to play multiple games simultaneously, were constrained to perfect information environments. The reason for this is based on the intrinsic dynamics of both types of game environments. Perfect information games, like Chess and Go, are a good fit for ML techniques relying on self-play learning and local min-max search of the gamespace, while imperfect information games like Poker rely on game-reasoning techniques. Earlier this week, DeepMind unveiled a new ML method that can change these constraints forever.
Player of Games (PoG) is the first ML model able to play perfect and imperfect information games at scale. Created by DeepMind, PoG combines self-play learning, search, and game-theoretic reasoning in a single model that can adapt to perfect and imperfect information environments. The model achieved super-human performance in perfect games like Chess and Go as well as imperfect games like Poker or Scotland Yard. Beyond the applications in gaming, PoG is a massive leap towards building ML models that can adapt to real-world domains such as weather forecasting or energy optimization that combine perfect and imperfect information. We should expect PoG to become a seminal algorithm in the next wave of ML gaming research.
🔺🔻 TheSequence Scope is our Sunday free digest. To receive high-quality educational content about the most relevant concepts, research papers and developments in the ML world every Tuesday and Thursday, please subscribe to TheSequence Edge 🔺🔻
🗓 Next week in TheSequence Edge:
Edge#149: we discuss Model Tracing and Lineage; we explore MLTrace, a reference architecture for observability in ML pipelines; we overview M3, a platform that powers time-series at Uber.
Edge#150: we deep dive into Microsoft’s SynapseML, a new framework for large-scale ML.
Now, let’s review the most important developments in the AI industry this week
🔎 ML Research
Playing Perfect and Imperfect Games
DeepMind published a paper detailing Player of Games, an algorithm that can play both perfect and imperfect information games →read more in the original research paper
Language Models at Scale
DeepMind published three papers detailing new techniques to scale language models →read more on DeepMind blog
Debugging ML Models
Amazon Research published a paper proposing a method for debugging ML models →read more on Amazon blog
Improving Transformers for Computer Vision
Google Research published a paper proposing a technique to optimize transformers for computer vision by using a smaller number of tokens →read more on Google Research blog
🤖 Cool AI Tech Releases
MiniTorch
The code for MiniTorch, a library for learning the principles of ML in Python, was open-sourced →read more on MiniTorch’s Github
🛠 Real World ML
Testing Firefox
Mozilla published a blog post detailing the ML architecture they use to test Firefox more efficiently →read more on Mozilla blog
LinkedIn Analytics Stack
LinkedIn published a blog post describing the evolution of their analytics stack →read more on LinkedIn blog
Large Language Models for Shopping Recommendations
Korean search giant Naver is using massive language pretrained models to power shopping recommendations →read more in this article from VentureBeat
🗯 Useful Tweet

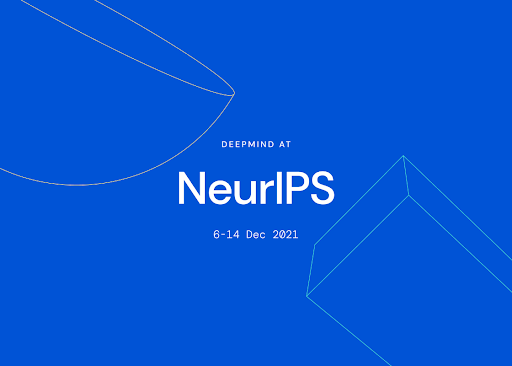
💸 Money in AI
ML&AI
Development platform for embedded ML Edge Impulse raised $34 million in a Series B round led by Coatue. Hiring remote.
Model vulnerabilities detection and prevention startup Robust Intelligence raised a $30 million Series B funding round led by Tiger Global. Hiring in San Francisco/US.
AI-powered
Voice platform for healthcare professionals Suki raised $55 million in a Series C round of funding led by March Capital. Hiring in Bengaluru/India and Redwood City/US.
Talent engagement platform Sense raised $50 million in series D funding led by SoftBank. Hiring in Bengaluru/India and San Francisco/US.
AI video generation platform Synthesia raised $50 million in a Series B round led by Kleiner Perkins. Hiring globally.
Customer engagement platform MoEngage raised a $30 million Series D financing round led by Steadview Capital. Hiring globally.
Conversational AI company Posh Technologies raised $27.5 million in Series A funding led by Canapi Ventures. Hiring in Boston/US.
Cloud analytics application platform NetSpring raised $13 million in a Series A round led by Dell Technologies Capital. Hiring in San Francisco/US.